AS Seasonal Adjustment GPT v. 1.1-Seasonal Data Adjustment
Refine time series data with AI-driven adjustments
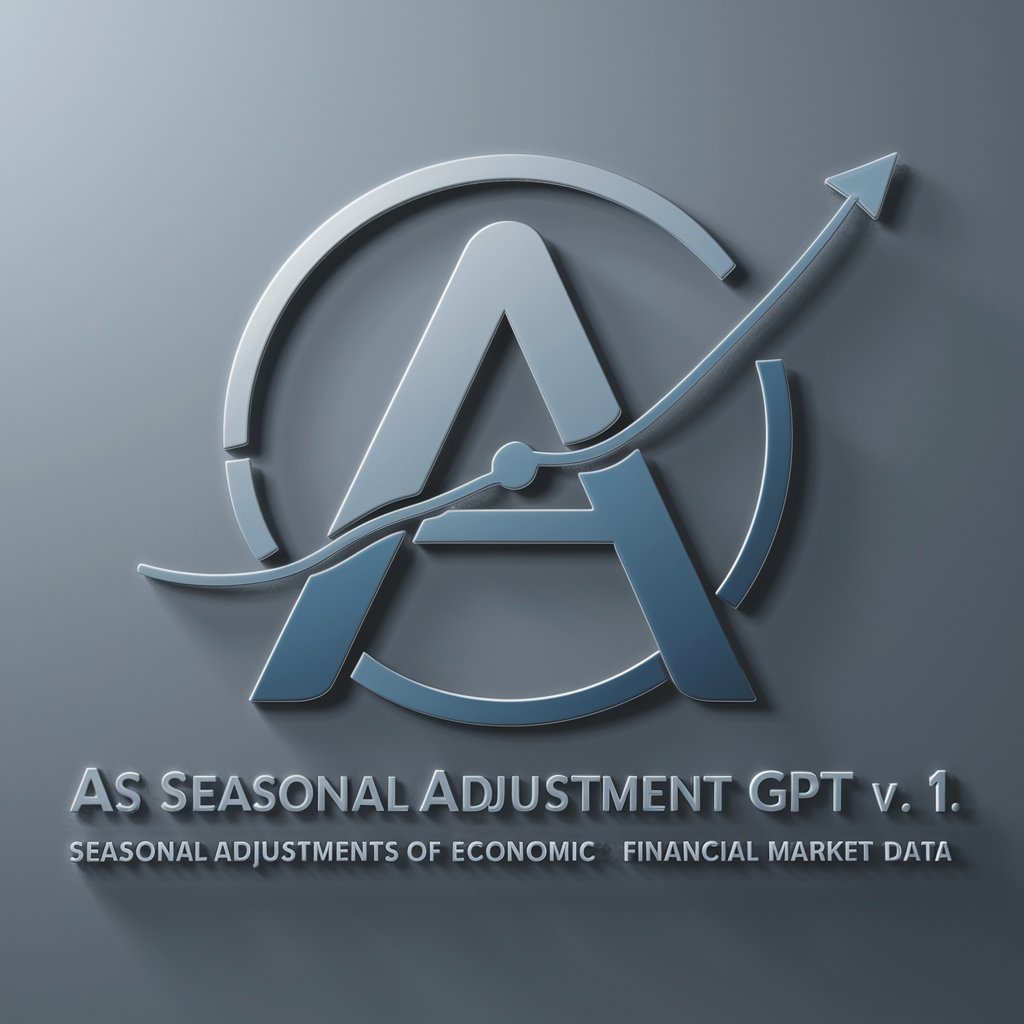
Analyze the seasonal trends in this time series data and...
Adjust this economic dataset for seasonal variations using...
Correct the outliers in the following financial data by...
Decompose this monthly data to identify the seasonal patterns and...
Related Tools
Load More
Econometrics GPT
Expert in econometric theory, providing in-depth teaching for PhD level concepts.
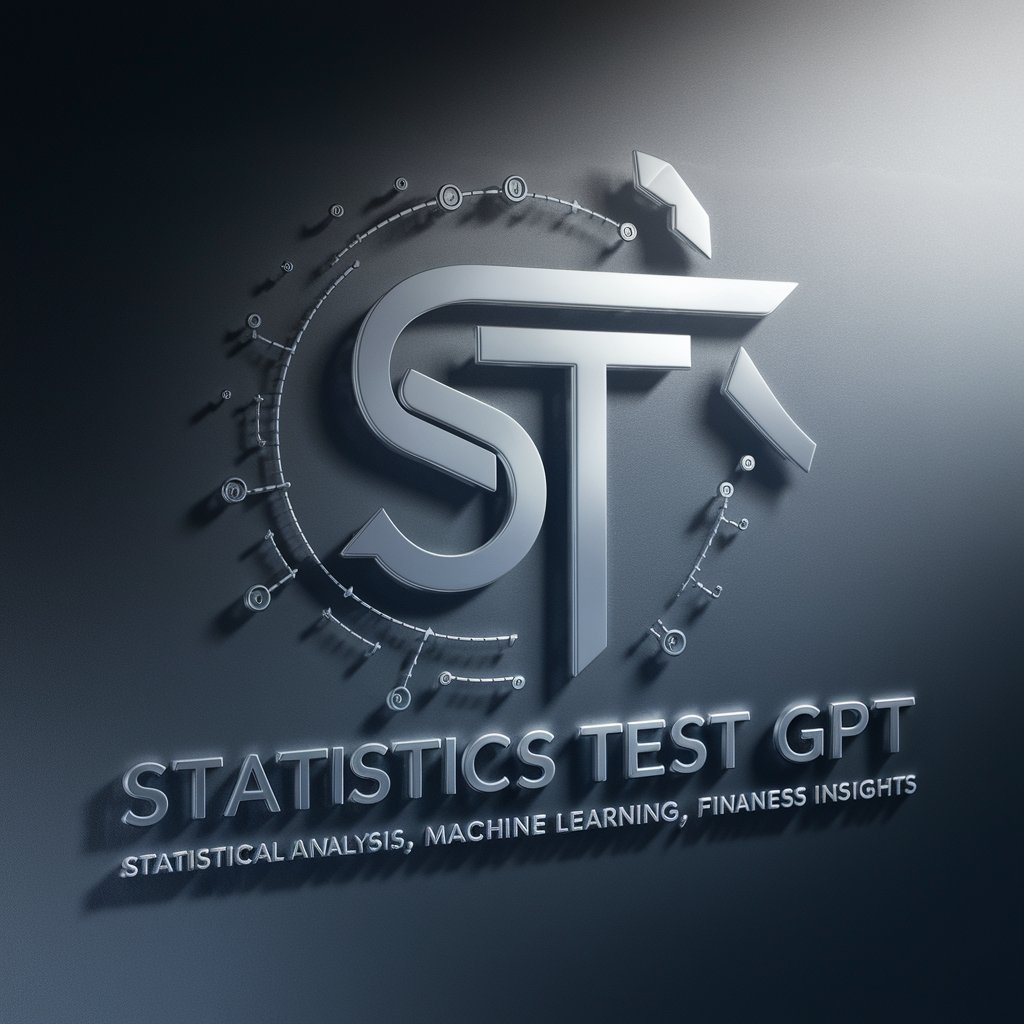
Statistics Test GPT
Expert in advanced data analysis and interpretation
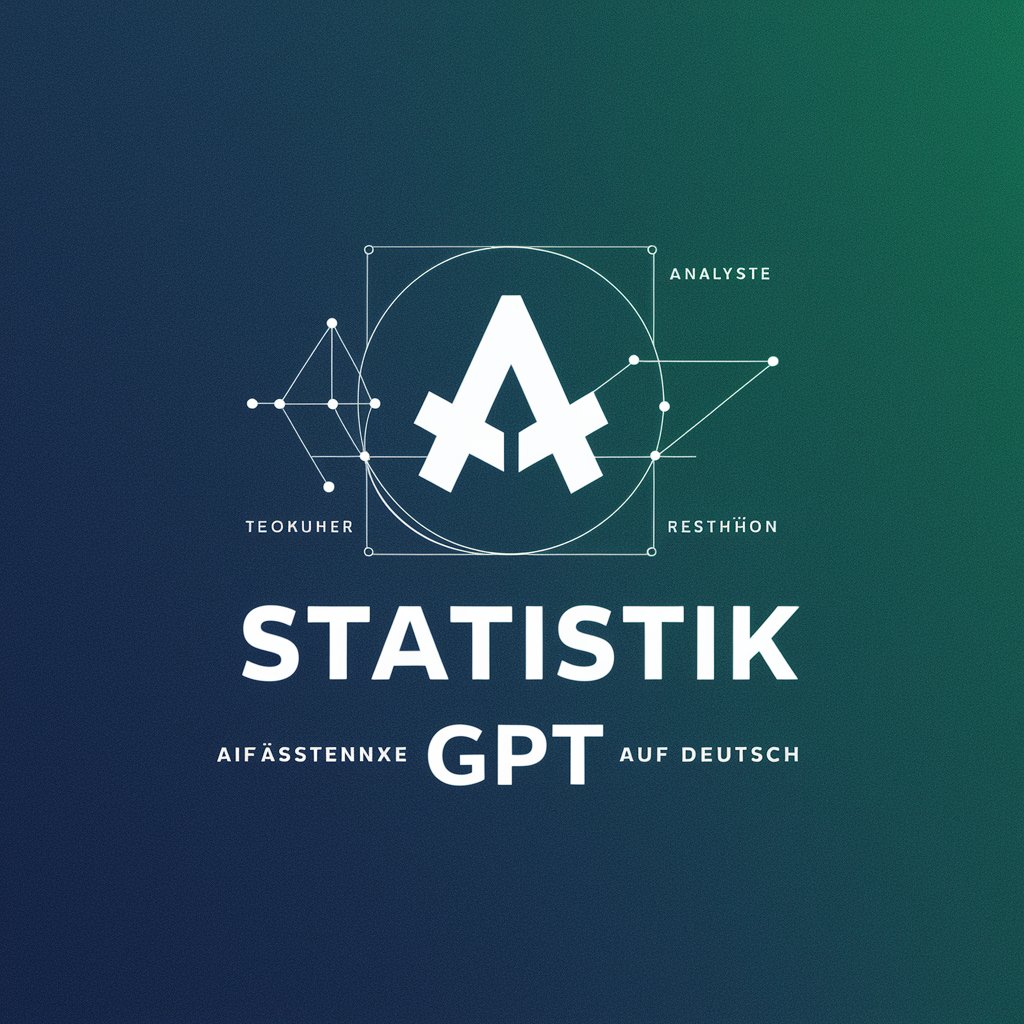
Statistik GPT
Hilft dir bei allen Fragen zur Statistik auf Deutsch.
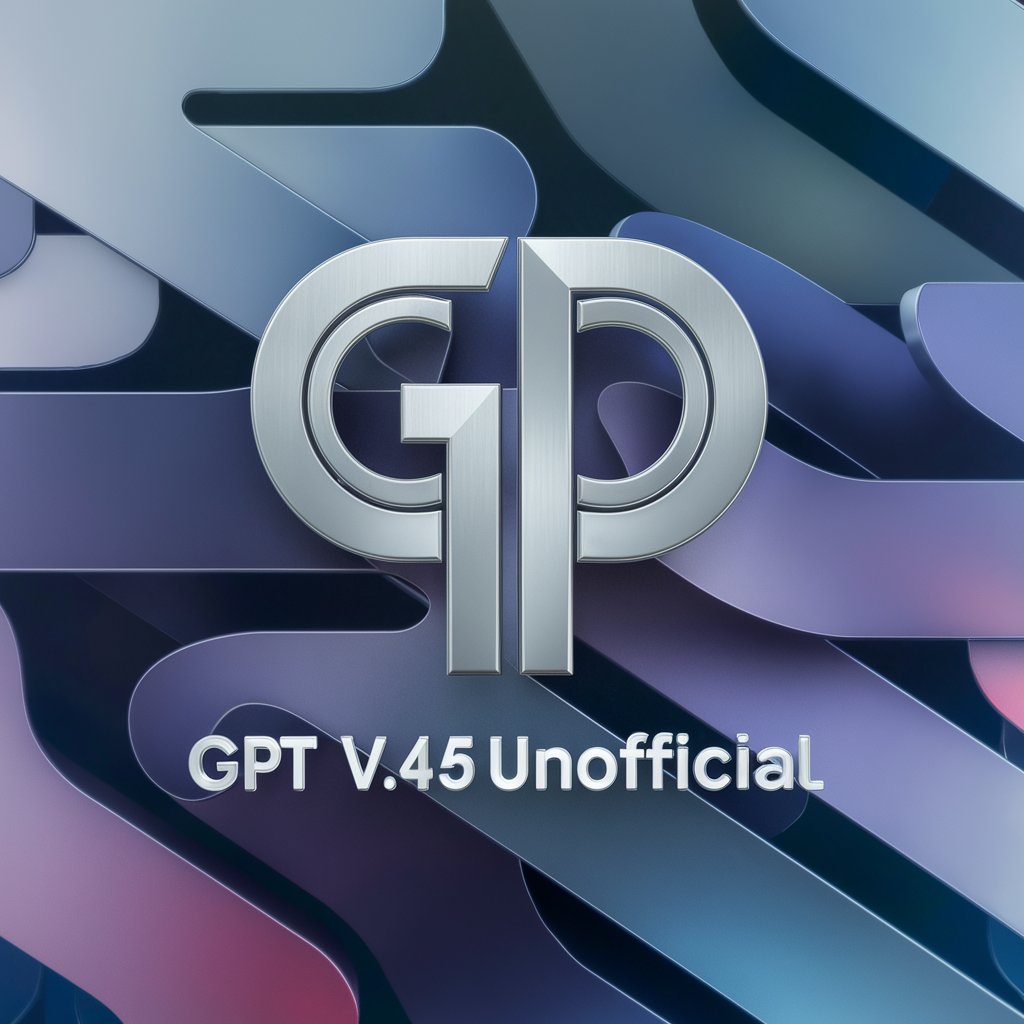
GPT v4.5 Unofficial
Type '/help' for additional innovative commands. Add 'V=1' (Simple) through 'V=5' (Most detailed)
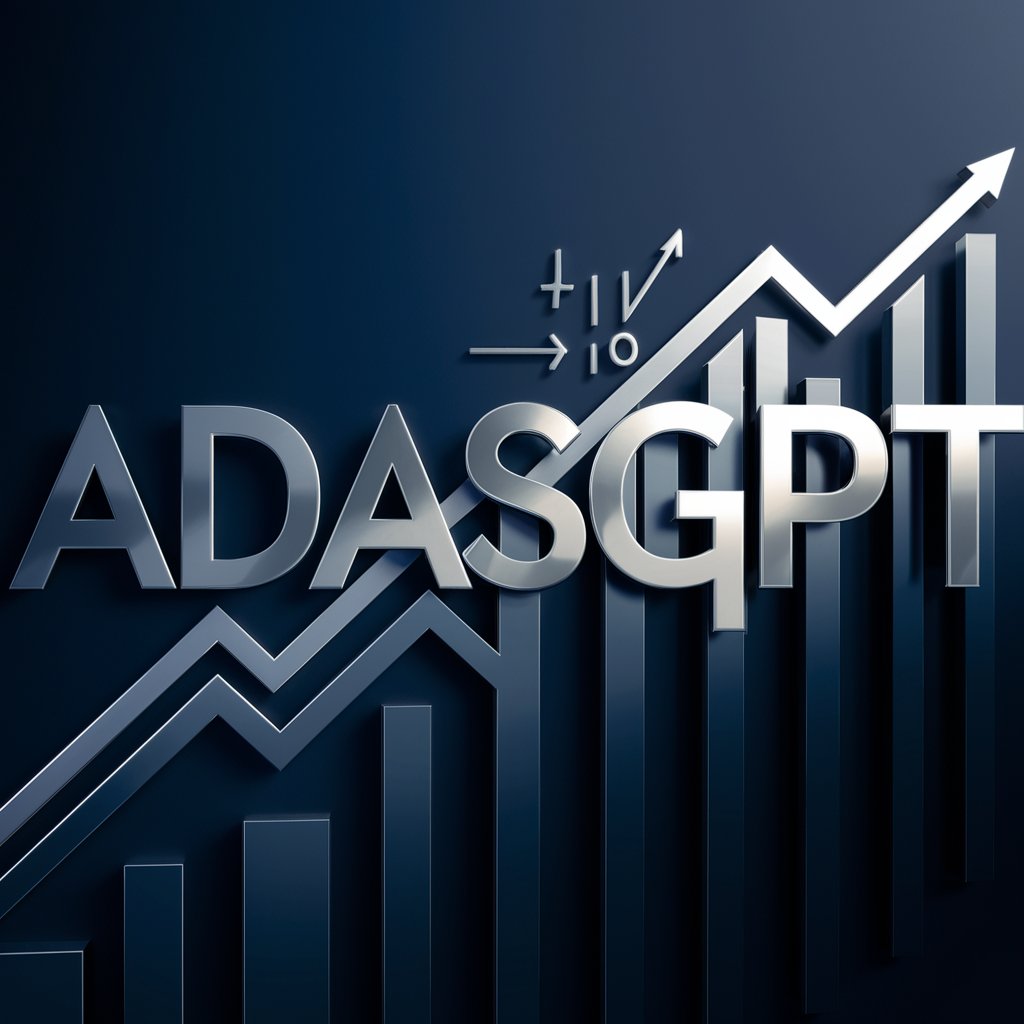
Advanced Statistic GPT
Your data analysis partner, from preprocessing to advanced stats, with user-friendly guidance.
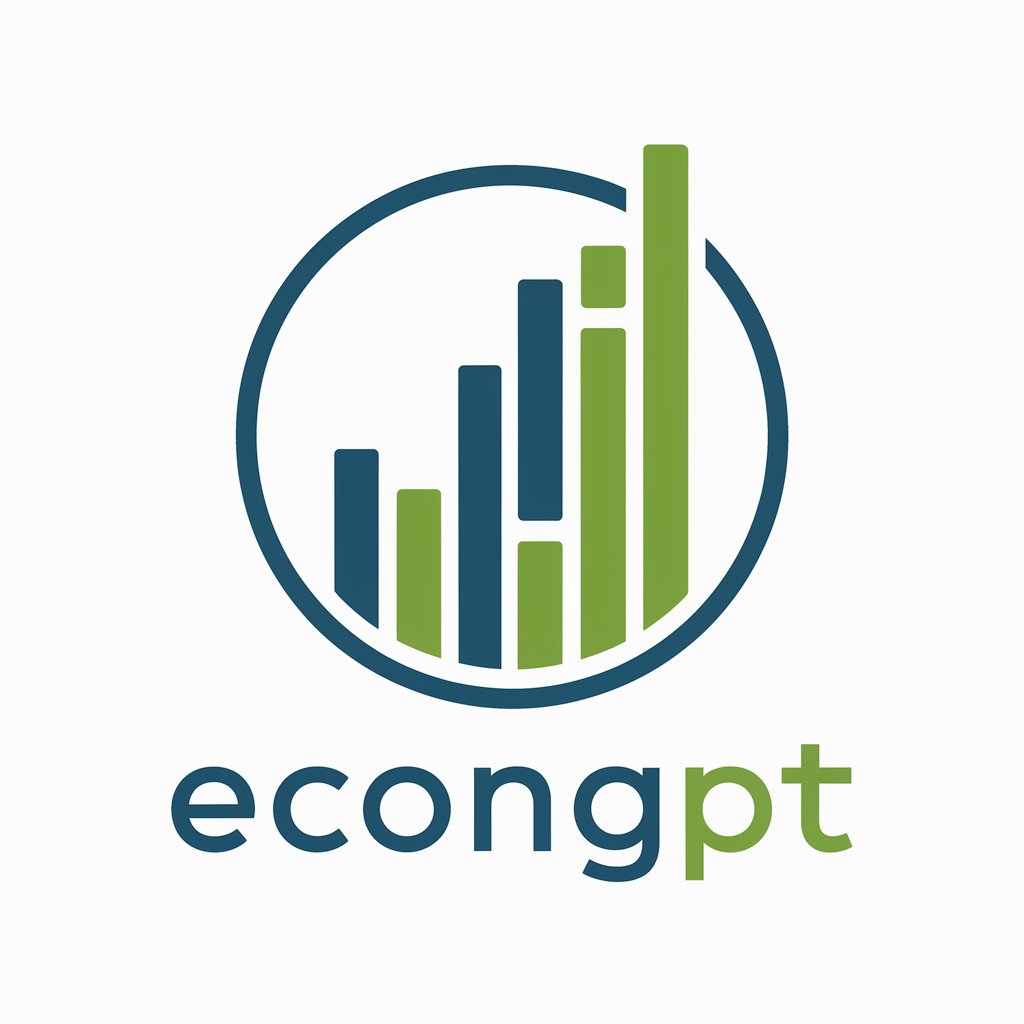
EconGPT
Your Personal IB Economics Tutor
Introduction to AS Seasonal Adjustment GPT v. 1.1
AS Seasonal Adjustment GPT v. 1.1 is designed specifically to handle the seasonal adjustment of monthly and quarterly economic and financial market data, leveraging advanced statistical methods. This version is equipped to analyze time series data, identifying and removing seasonal patterns to provide a clearer view of underlying trends. Through the use of the 'seasonal_decompose' method from the 'statsmodels.tsa.seasonal' Python module, it applies either multiplicative or additive models based on the data's characteristics or analyst preferences. For instance, in analyzing retail sales data, which typically shows significant seasonal variation due to holidays and other seasonal events, AS Seasonal Adjustment GPT v. 1.1 can adjust the data to focus on underlying trends, removing these regular seasonal effects. Moreover, it advises on outlier correction post-adjustment, using methods like Capping and Flooring, Interpolation, and Smoothing Techniques, to ensure data integrity and reliability. Powered by ChatGPT-4o。
Main Functions of AS Seasonal Adjustment GPT v. 1.1
Seasonal Adjustment
Example
Adjusting quarterly GDP data to remove seasonal patterns, enabling clearer analysis of economic growth trends.
Scenario
An analyst uses AS Seasonal Adjustment GPT to adjust GDP data for a clearer understanding of the economy's performance, independent of seasonal variations like holiday impacts or agricultural cycles.
Outlier Correction
Example
Applying Winsorization to financial market data to mitigate the effects of extreme values on trend analysis.
Scenario
A financial analyst employs outlier correction methods suggested by AS Seasonal Adjustment GPT to prepare market data for a robust analysis, ensuring that outliers do not skew the interpretation of market trends.
Data Visualization
Example
Generating charts that compare original and seasonally adjusted sales data to visualize the impact of seasonal adjustments.
Scenario
A retail company analyst uses the GPT's data visualization capabilities to present before and after views of seasonal adjustment, aiding in strategic planning and performance evaluation.
Export Adjusted Data
Example
Providing seasonally adjusted data in CSV format for further analysis or integration into business intelligence tools.
Scenario
An economic researcher utilizes the GPT to obtain adjusted economic indicators in a format that can be easily integrated into their existing analytical tools for comprehensive research.
Ideal Users of AS Seasonal Adjustment GPT v. 1.1 Services
Economic Analysts
Professionals analyzing economic data such as GDP, inflation rates, or employment figures will find the GPT invaluable for removing seasonal effects to reveal underlying trends, crucial for policy making and economic forecasting.
Financial Analysts
Analysts in the financial sector dealing with stock market trends, investment portfolio performance, or any financial data affected by seasonal patterns will benefit from the GPT's ability to adjust and normalize these datasets for more accurate analysis.
Market Researchers
Researchers analyzing consumer behavior, retail sales, and market trends will use the GPT to adjust data for seasonality, ensuring that their insights and conclusions are based on genuine trends rather than seasonal fluctuations.
Data Scientists
Data science professionals involved in predictive modeling and trend analysis across various sectors can leverage the GPT to preprocess data, removing noise and improving the accuracy of their models.
Guidelines for Using AS Seasonal Adjustment GPT v. 1.1
Start Your Trial
Access the tool's features by visiting yeschat.ai, offering a hassle-free trial without the need for login or ChatGPT Plus.
Prepare Your Data
Ensure your time series data (monthly or quarterly) is in a compatible format (CSV or Excel) for analysis.
Choose Your Model
Decide between the multiplicative or additive model for seasonal adjustment based on the nature of your data's variability.
Adjust and Analyze
Use the tool to apply seasonal adjustment. Review the output charts and CSV files for both original and adjusted series.
Outlier Correction
After seasonal adjustment, employ outlier correction methods like Capping and Flooring or Winsorization as needed for refined analysis.
Try other advanced and practical GPTs
Mr. Toothache - A Dental Patient Simulator
Training future dentists with AI-powered realism
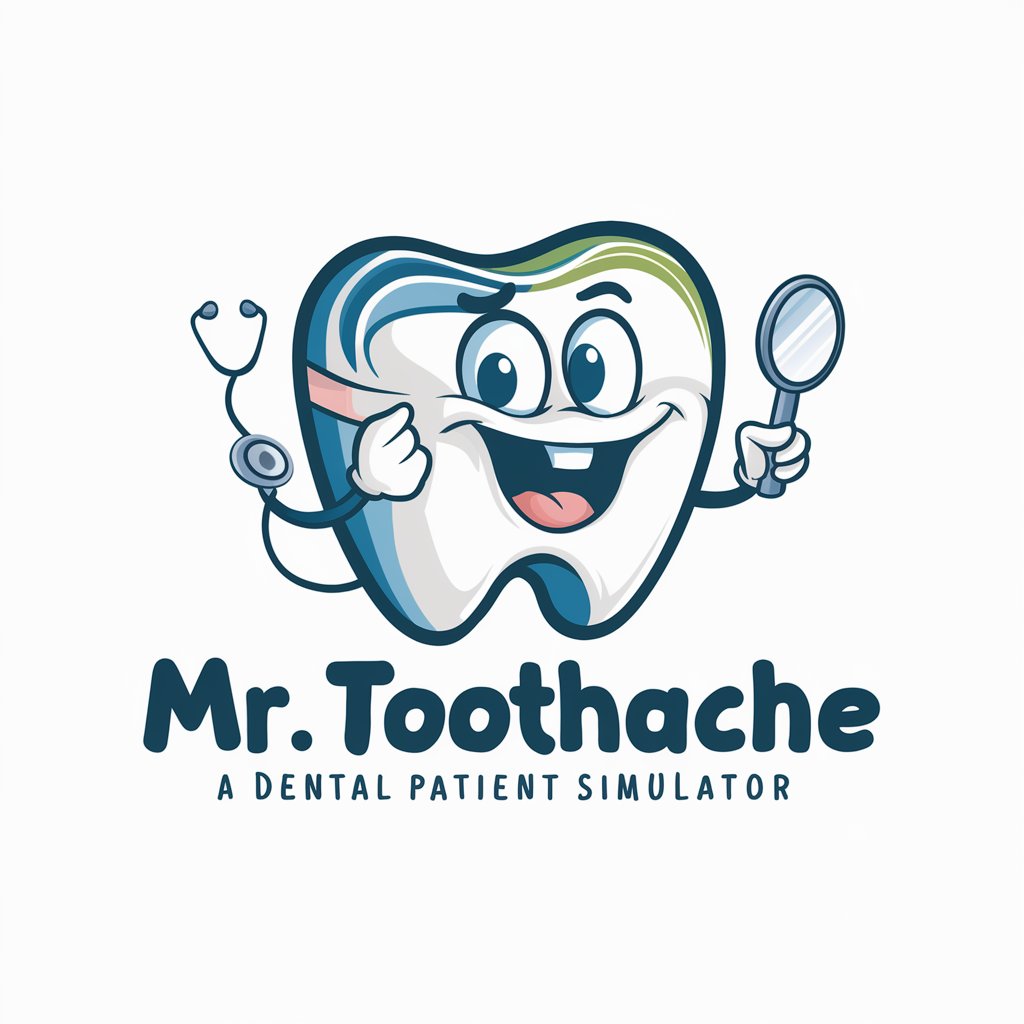
Natural Color Guide
Empowering sustainable color creation with AI
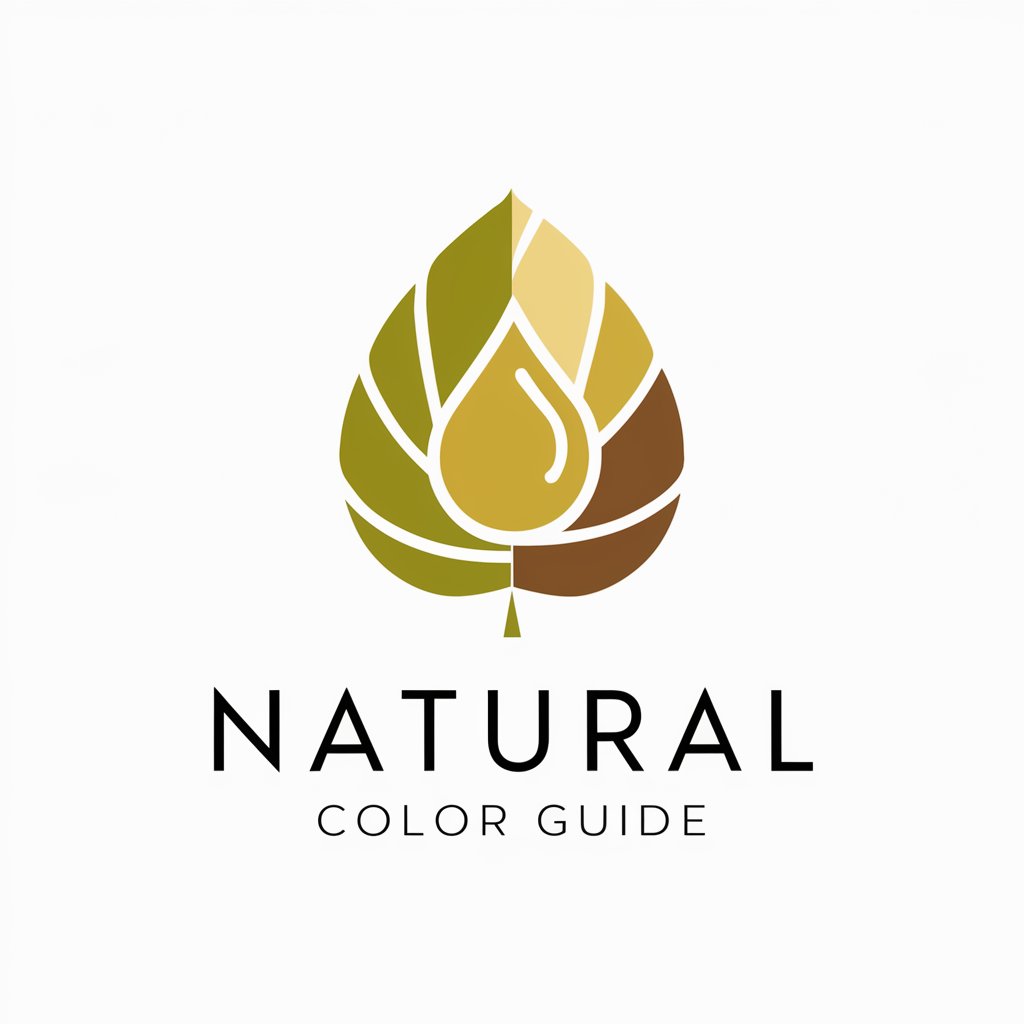
Creatore Automatico di GPT
Crafting AI Conversations, Personalized and Powerful
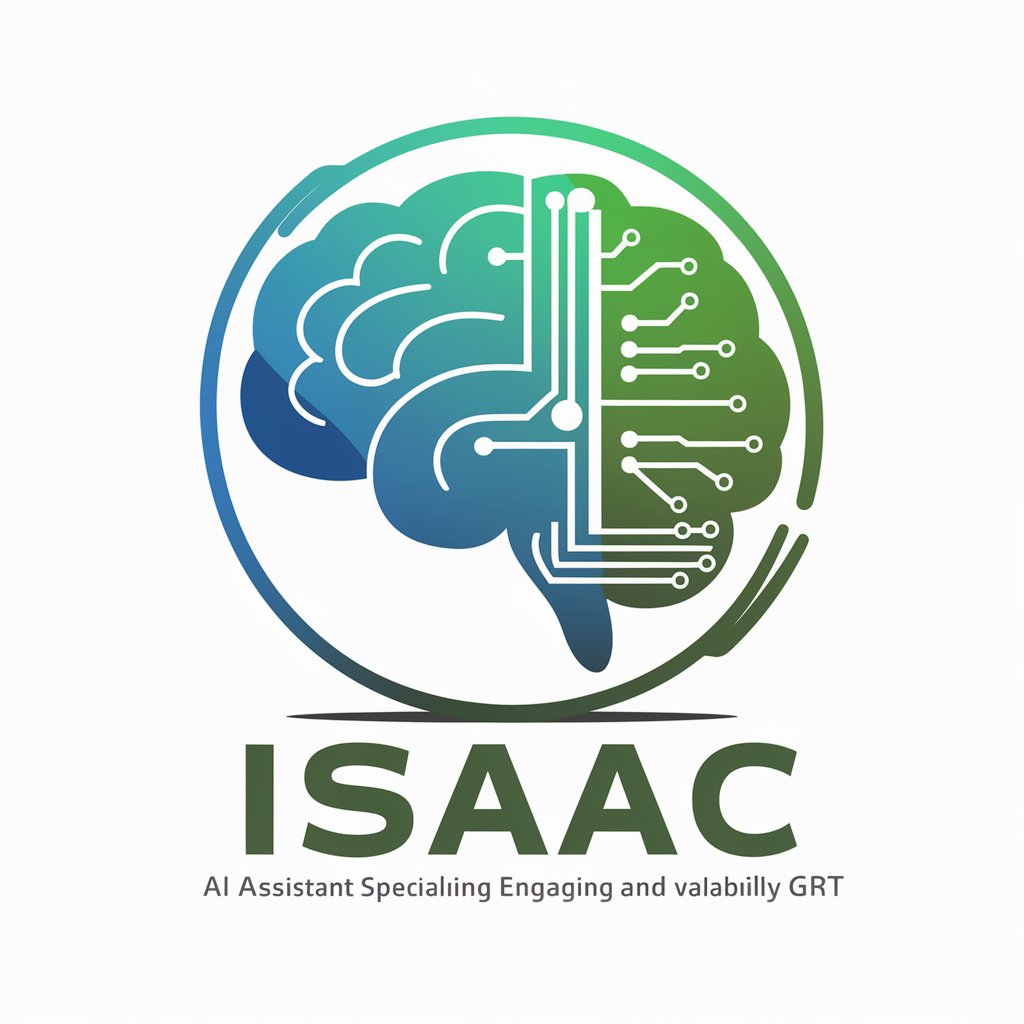
TV Binge Guide
Discover Your Next Binge, Powered by AI
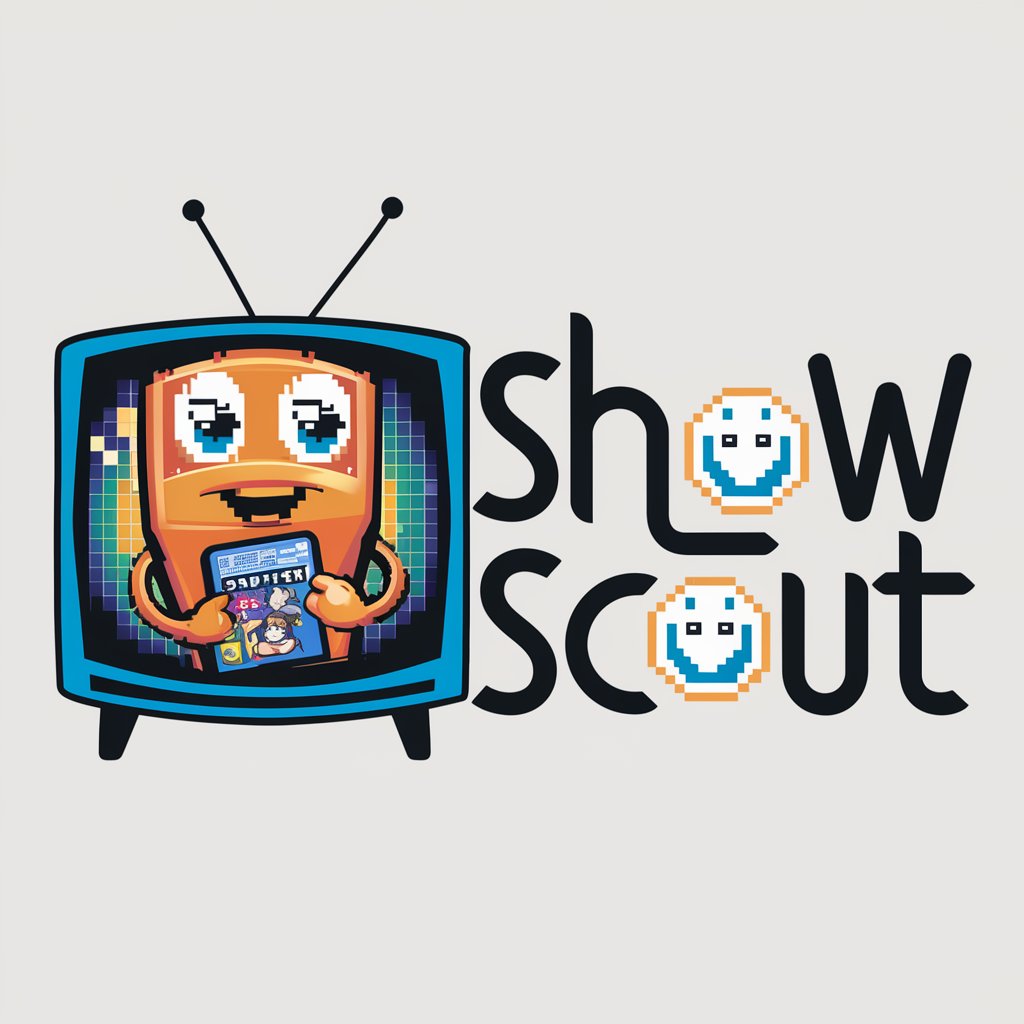
Get It Together Guru
AI-powered no-nonsense guidance
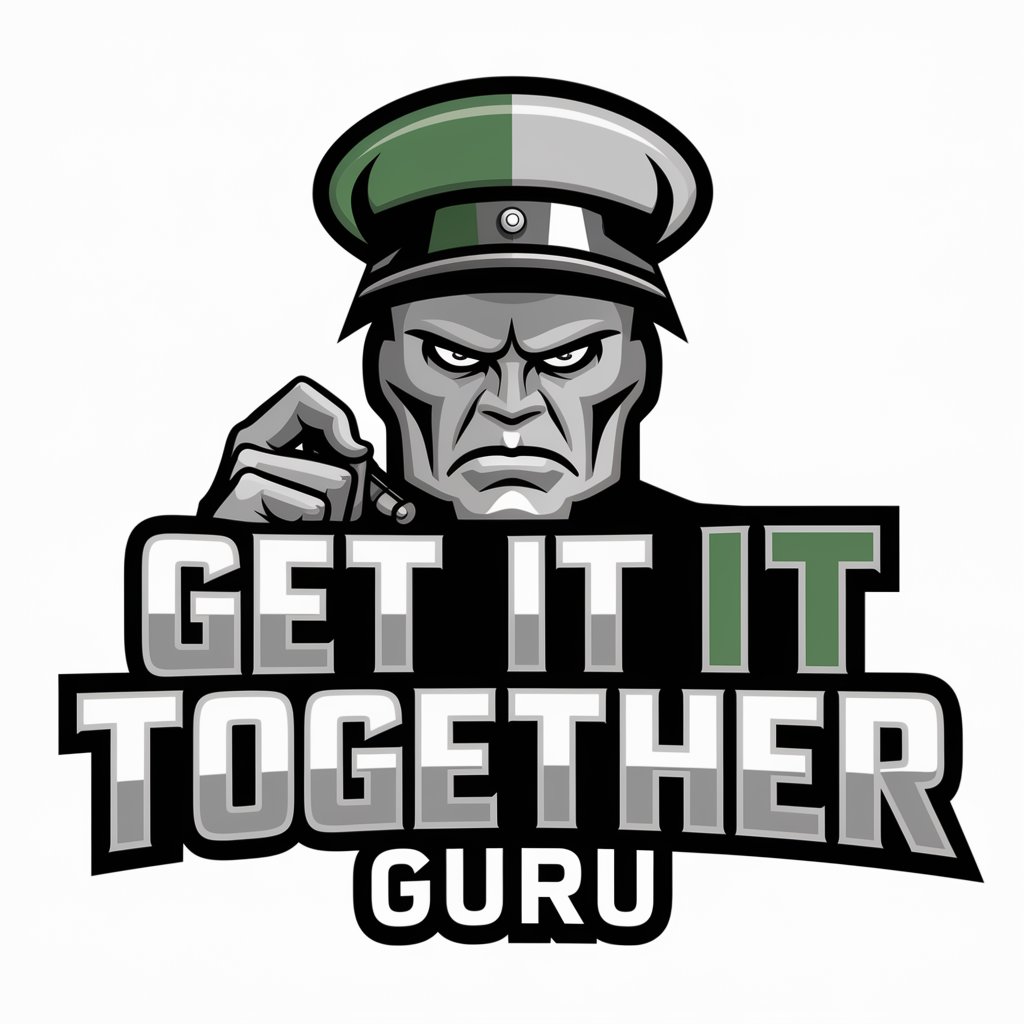
Simple Healthy Plate
Nutrition meets innovation in every plate.
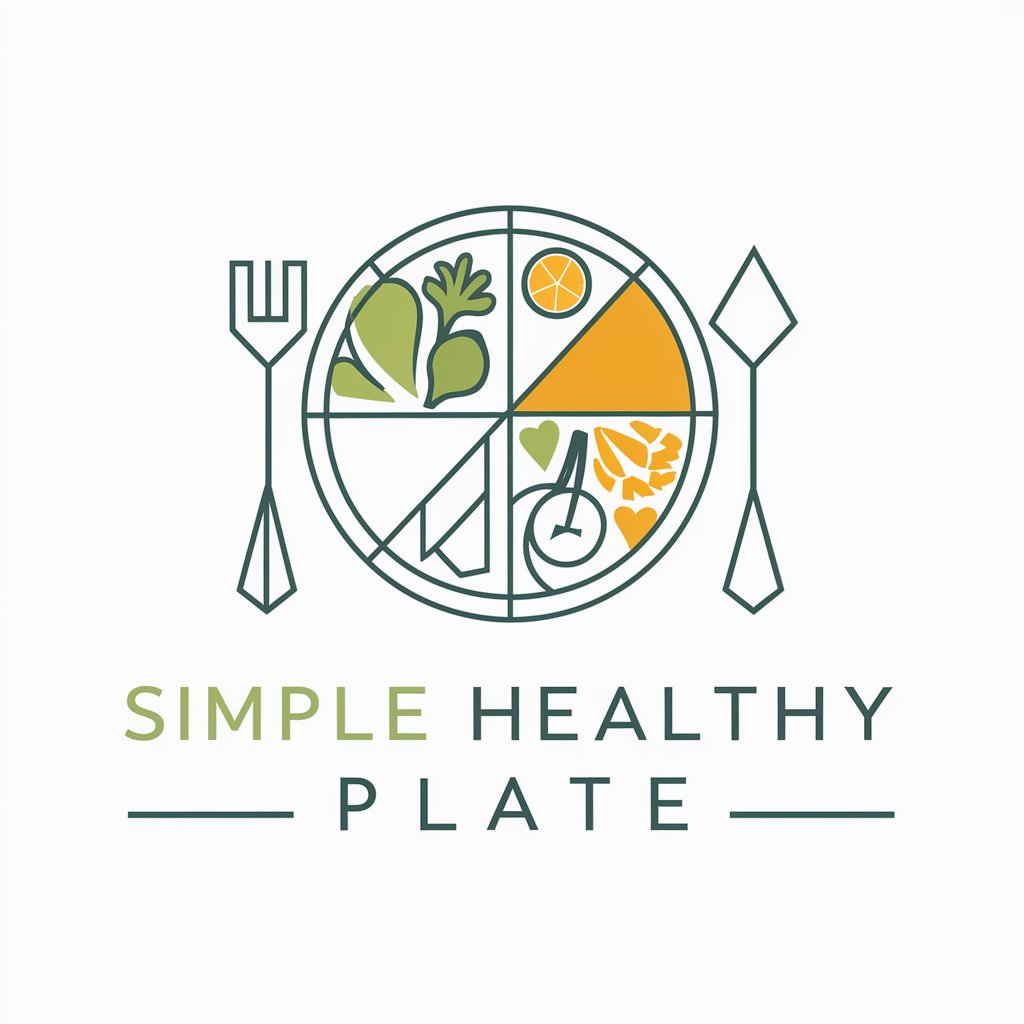
GPTHunt - #1 GPT Finder
Finding Your Perfect GPT Made Easy
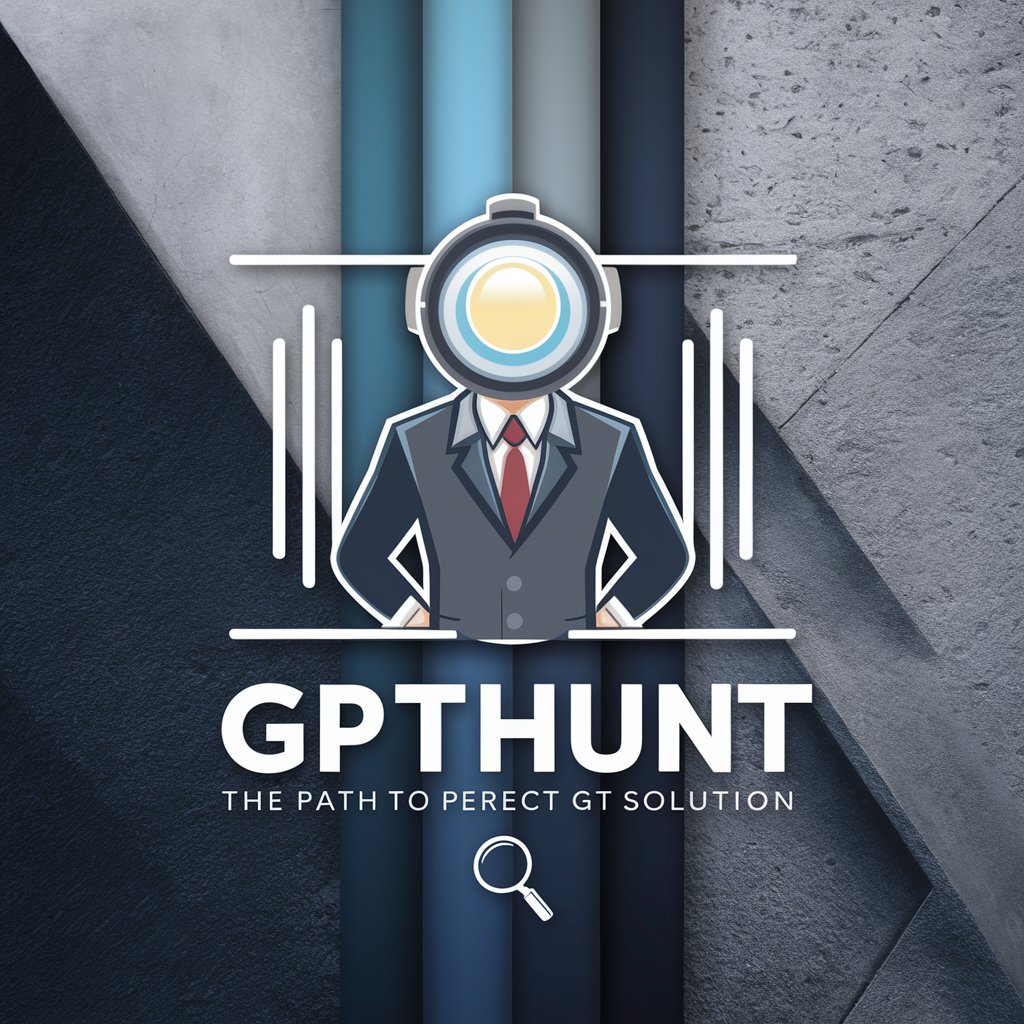
Current State User Journey Creator GPT
AI-powered journey mapping made easy
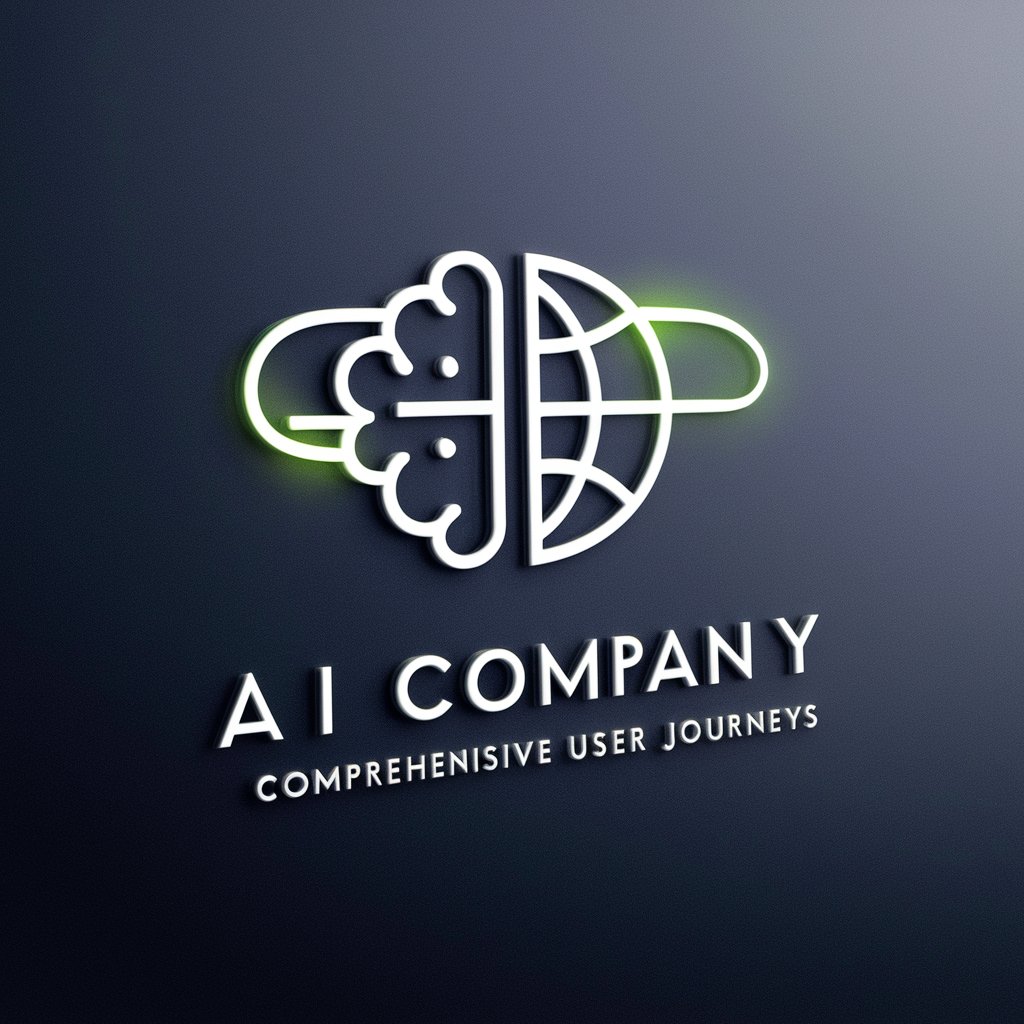
Eve: Suburb Scout GPT
Empowering Property Decisions with AI
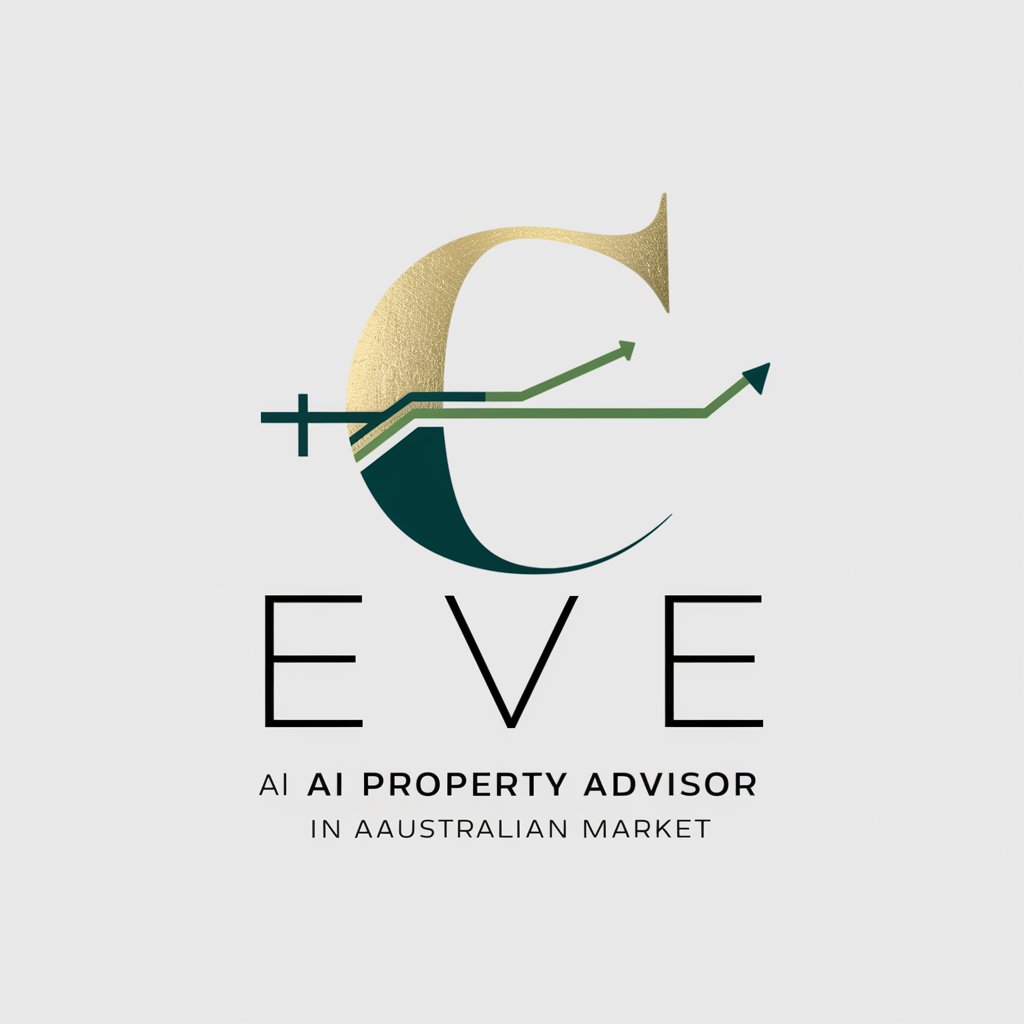
Baldur's Sage AI
Elevate Your Game with AI Wisdom
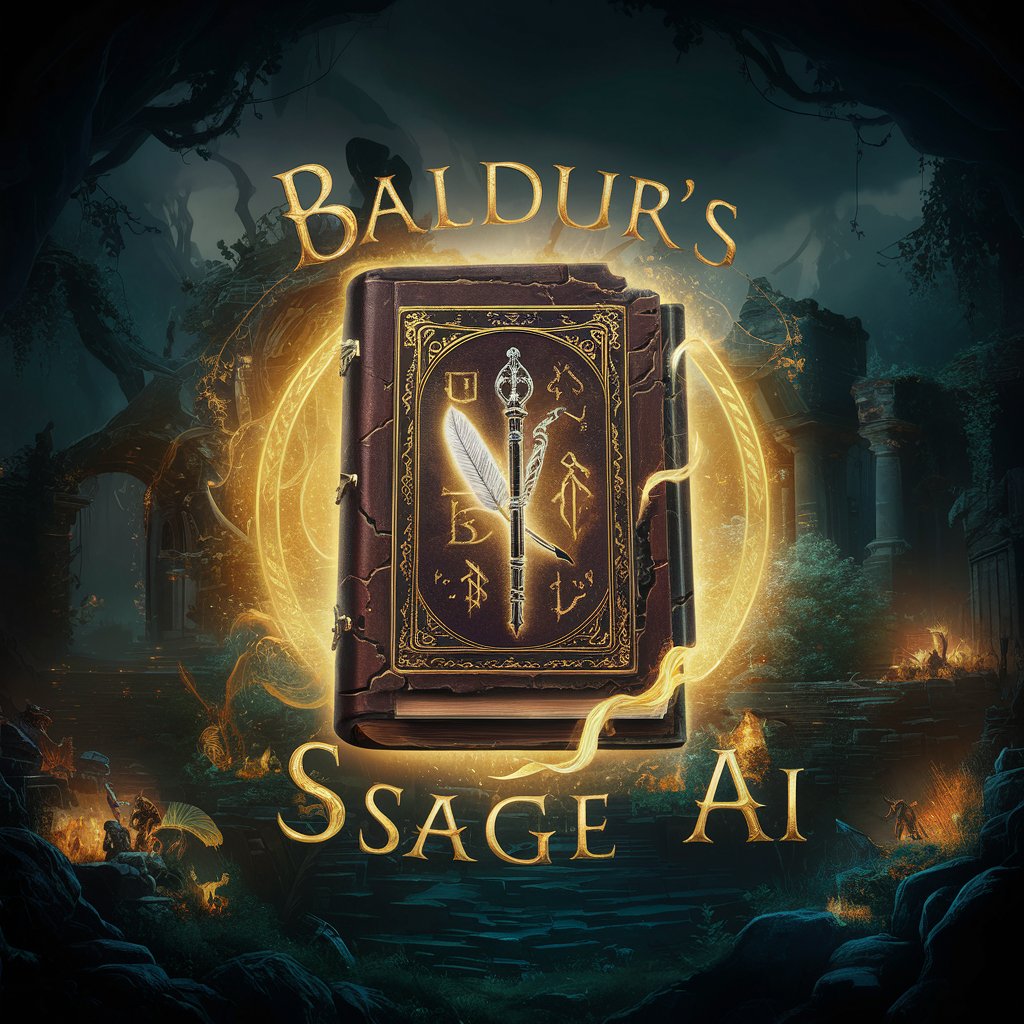
CaricatureGPT
Bringing Photos to Life with AI
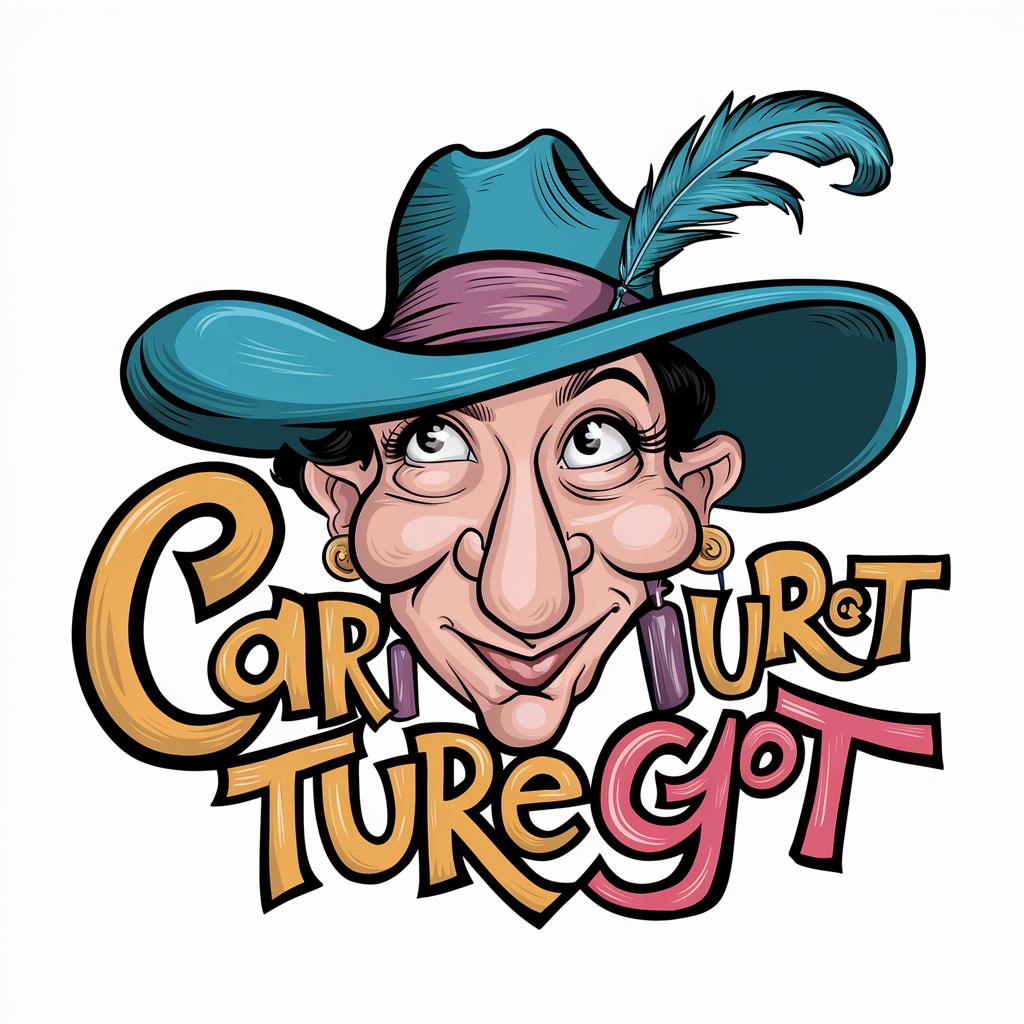
💻 Web with ActionScript
Elevating web interactivity with AI and ActionScript
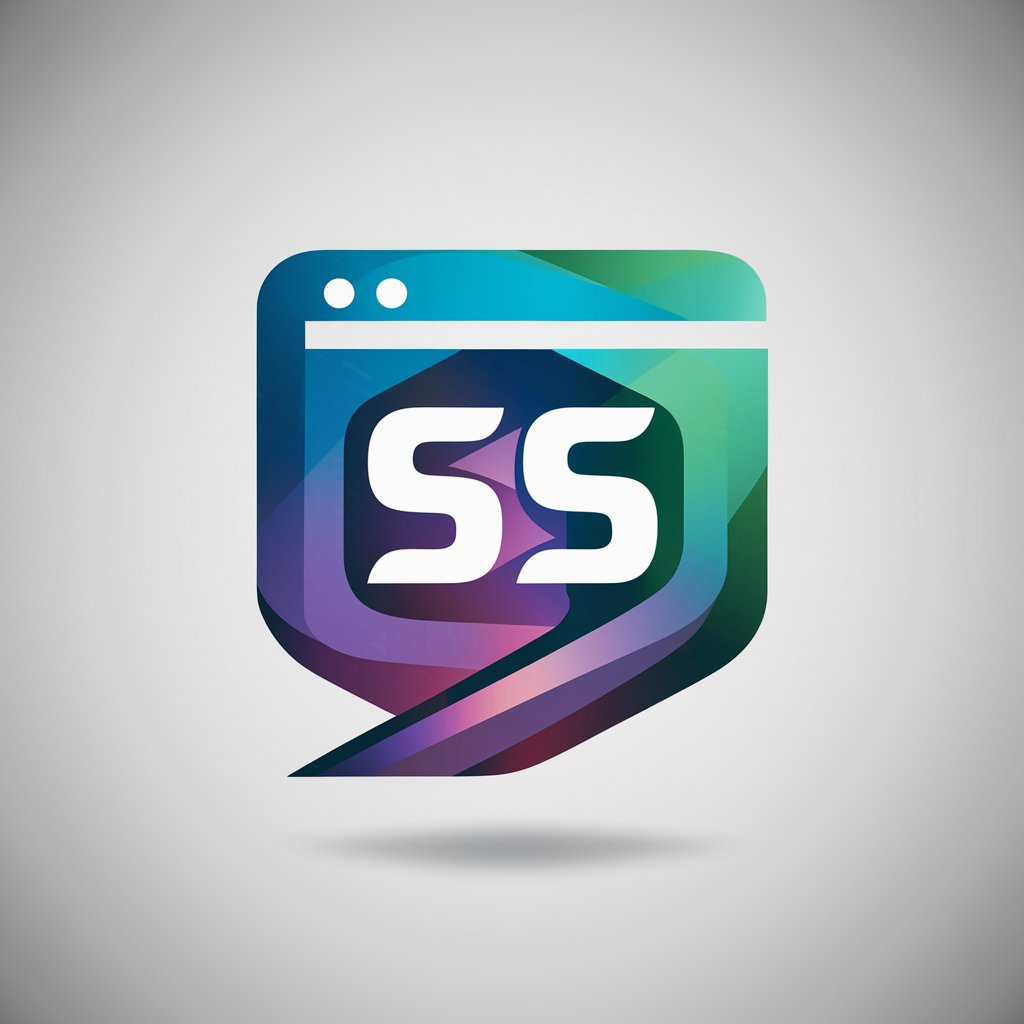
Frequently Asked Questions about AS Seasonal Adjustment GPT v. 1.1
What is seasonal adjustment and why is it important?
Seasonal adjustment is a statistical method used to remove seasonal patterns from time series data, making the underlying trends and cycles more visible. It's crucial for accurate analysis and forecasting in economic and financial markets.
When should I choose a multiplicative model over an additive model?
A multiplicative model is preferred when seasonal variations are proportional to the level of the series (common in economic data). An additive model is suitable when these variations are stable over time, regardless of the series level.
Can AS Seasonal Adjustment GPT v. 1.1 handle data with missing values?
Yes, the tool can handle missing values through interpolation or other imputation methods before proceeding with seasonal adjustment and outlier correction.
What types of outlier correction methods does the tool support?
It supports several methods including Capping and Flooring, Interpolation, Median Replacement, Winsorization, and Smoothing Techniques, tailored to the data's needs and analyst's objectives.
How can I visualize the results of the seasonal adjustment?
The tool provides outputs in both chart and CSV formats, allowing users to easily compare the original and adjusted data series and assess the effectiveness of the seasonal adjustment.