Interesting 'Attention is All You Need' - Transformer Model Exploration
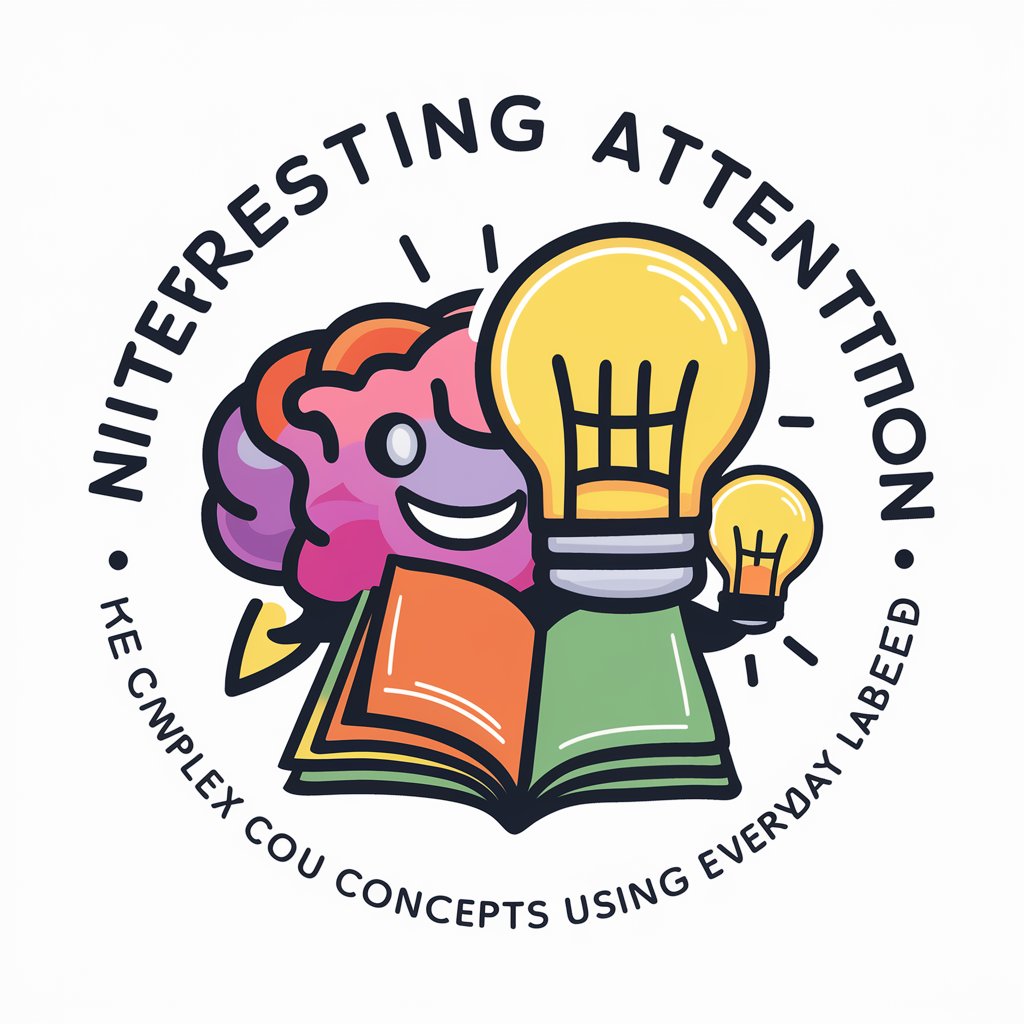
Hello! Ready to simplify complex ideas together?
Revolutionizing text processing with AI-powered attention.
Explain the concept of...
How does the 'Attention' mechanism...
Break down the idea of...
What is the role of...
Get Embed Code
Introduction to Interesting 'Attention Is All You Need'
Imagine you're at a busy party, trying to have a conversation with a friend. Despite the noise, your brain can focus on the conversation while ignoring irrelevant background noise. This ability to focus and draw connections is akin to the 'Attention Is All You Need' concept in machine learning. Our model, 'Interesting 'Attention Is All You Need'', simplifies this complex AI paper's ideas, making them accessible to everyone through relatable analogies and examples. Powered by ChatGPT-4o。
Main Functions and Use Cases
Simplifying complex AI concepts
Example
It's like translating a chef's sophisticated recipe into simple steps for a home cook.
Scenario
A student trying to grasp advanced AI concepts for their project can use our model to get clear, simple explanations.
Providing educational content
Example
Turning a dense academic textbook into an engaging comic book.
Scenario
A teacher explaining AI to young students uses our model to create engaging lessons with real-world analogies.
Assisting in research
Example
It's akin to a seasoned guide simplifying a complex jungle trail for novice hikers.
Scenario
An AI researcher can use our model to quickly understand new papers or concepts, saving time and effort.
Ideal User Groups
Students and Educators
This group includes anyone in an educational setting, from primary schools to universities, who might benefit from simplified explanations of complex AI concepts.
AI Enthusiasts and Hobbyists
Individuals with a keen interest in AI and machine learning, including self-learners and hobbyists, who appreciate accessible and engaging content.
Researchers and Professionals
AI professionals and researchers who need a quick and intuitive understanding of new concepts or technologies in the field.
How to Use Interesting 'Attention is All You Need'
Start your journey
Visit yeschat.ai for a free trial without login, and there's no need for ChatGPT Plus.
Understand the basics
Familiarize yourself with the core concepts of the Transformer model and attention mechanisms through the provided tutorials and documentation.
Experiment with the tool
Use the interactive examples to see how the Transformer model can be applied to various tasks such as translation, summarization, and question-answering.
Customize your experience
Explore customization options by tweaking model parameters or uploading your datasets to see how the model performs on your specific tasks.
Engage with the community
Join forums or community discussions to share your findings, ask questions, and get insights from other users.
Try other advanced and practical GPTs
Attention Hook Creator
Craft Compelling Hooks with AI
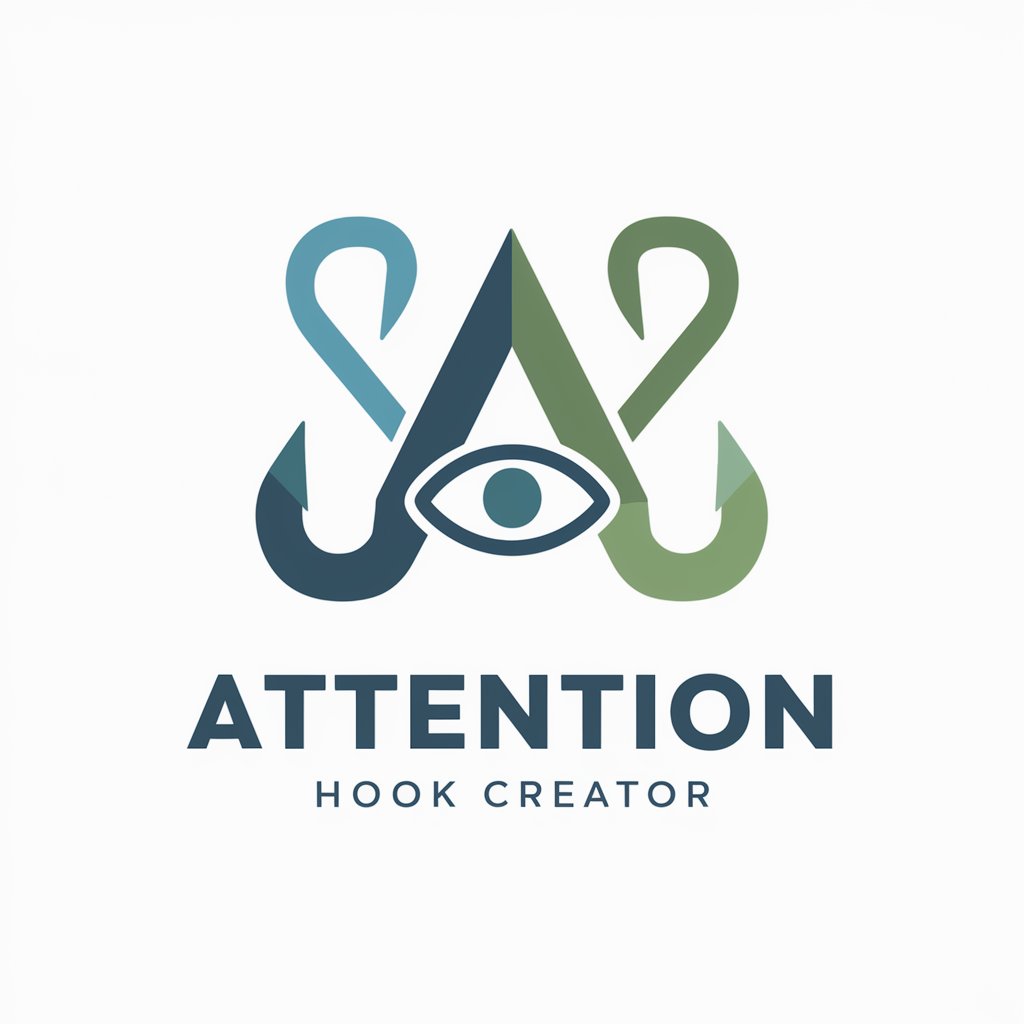
Tiny Creatures
Bringing your tiny ideas to life, artfully.
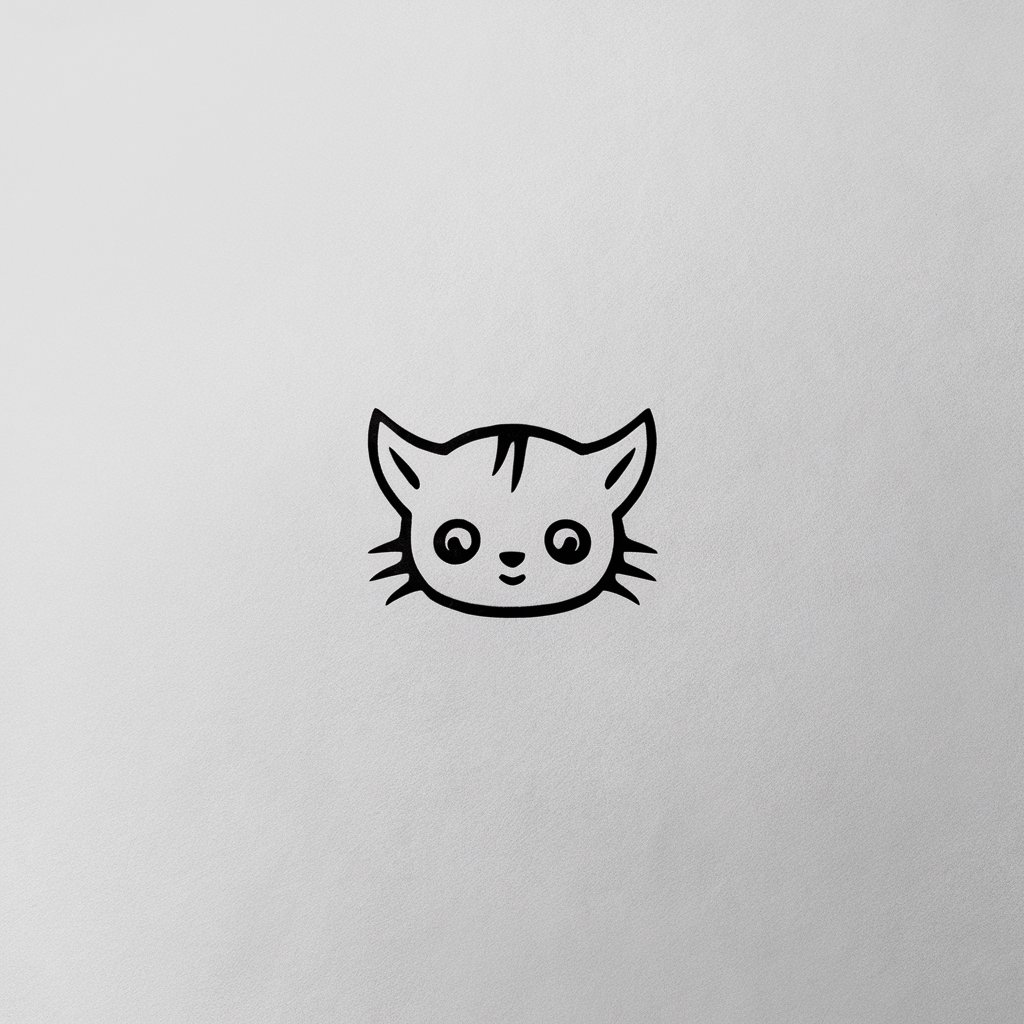
Creature Creator
Invent new species with AI imagination
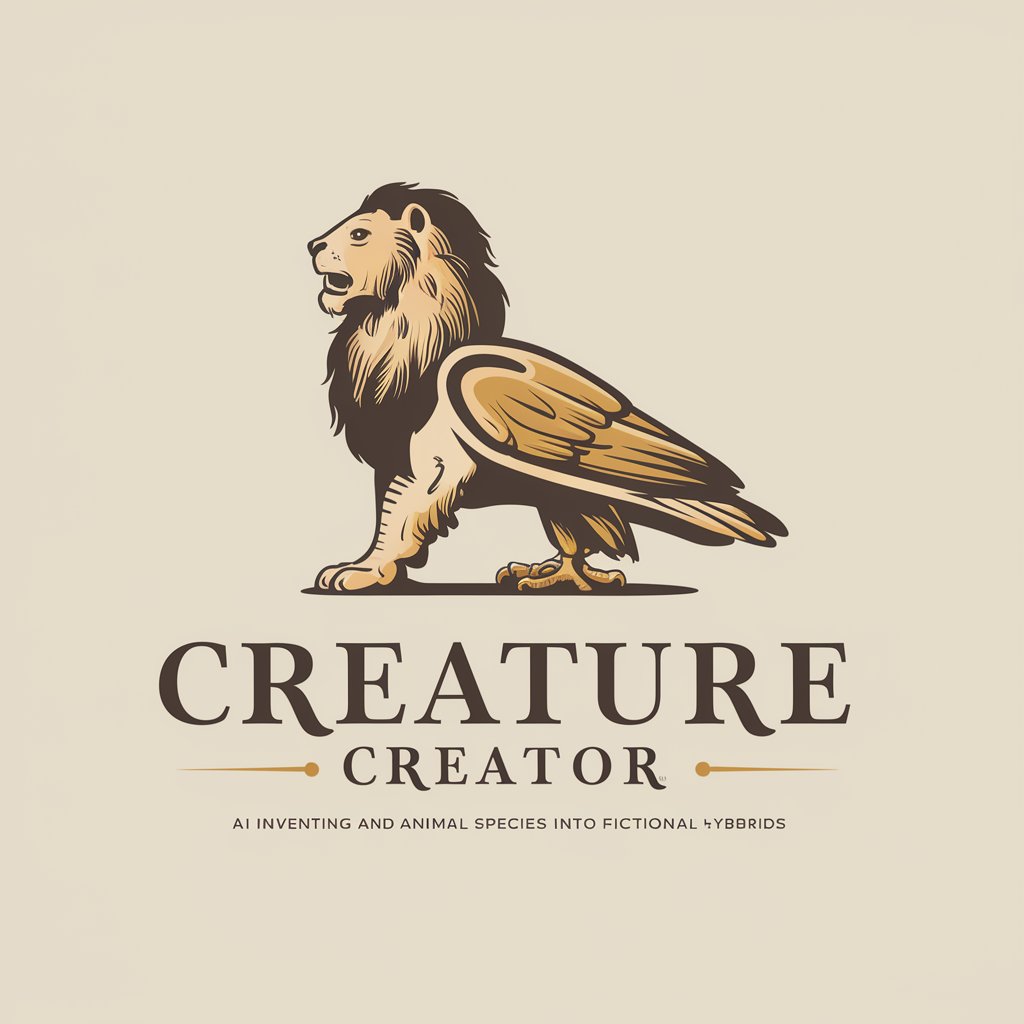
Curious Creature
Empowering curiosity with AI
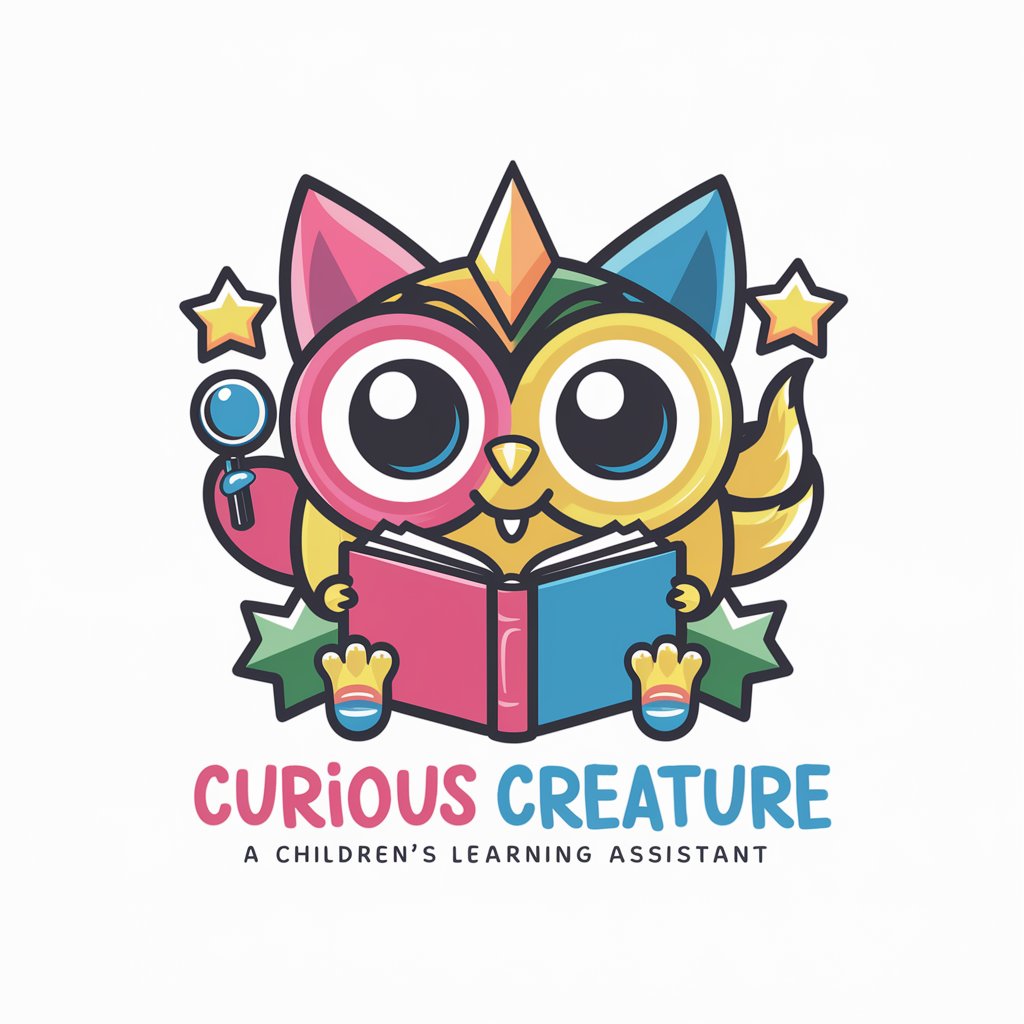
Cryptids & Mythical Creature Encounter Guide
Unveil the Mystical with AI-Powered Lore
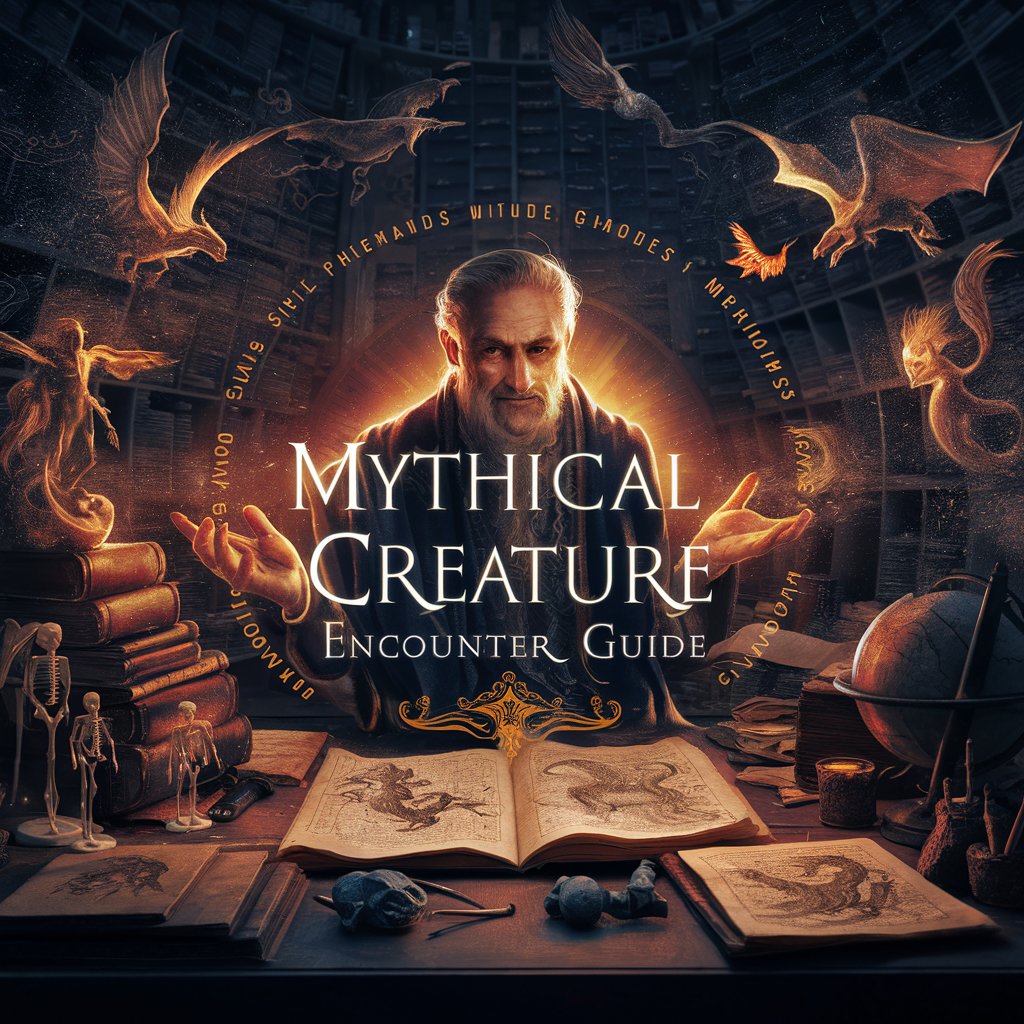
Creature Codex
Explore Myths with AI
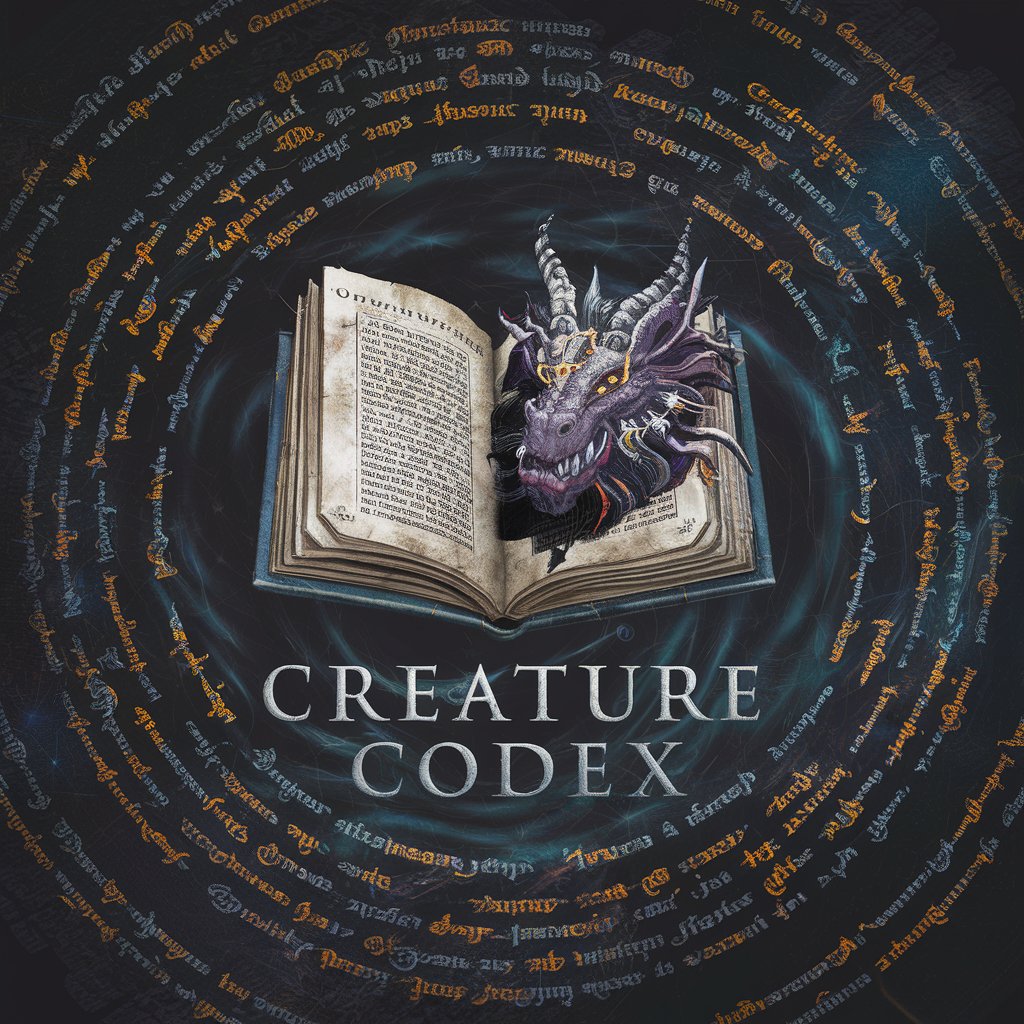
Allie - ADHD Attention Ally
Empowering ADHD management through AI conversation

Attention Grabbing Video Titles and One liners
Empower your videos with AI-driven creativity

Attention Hooker (Get hooks for your audience)
Capture attention, boost engagement
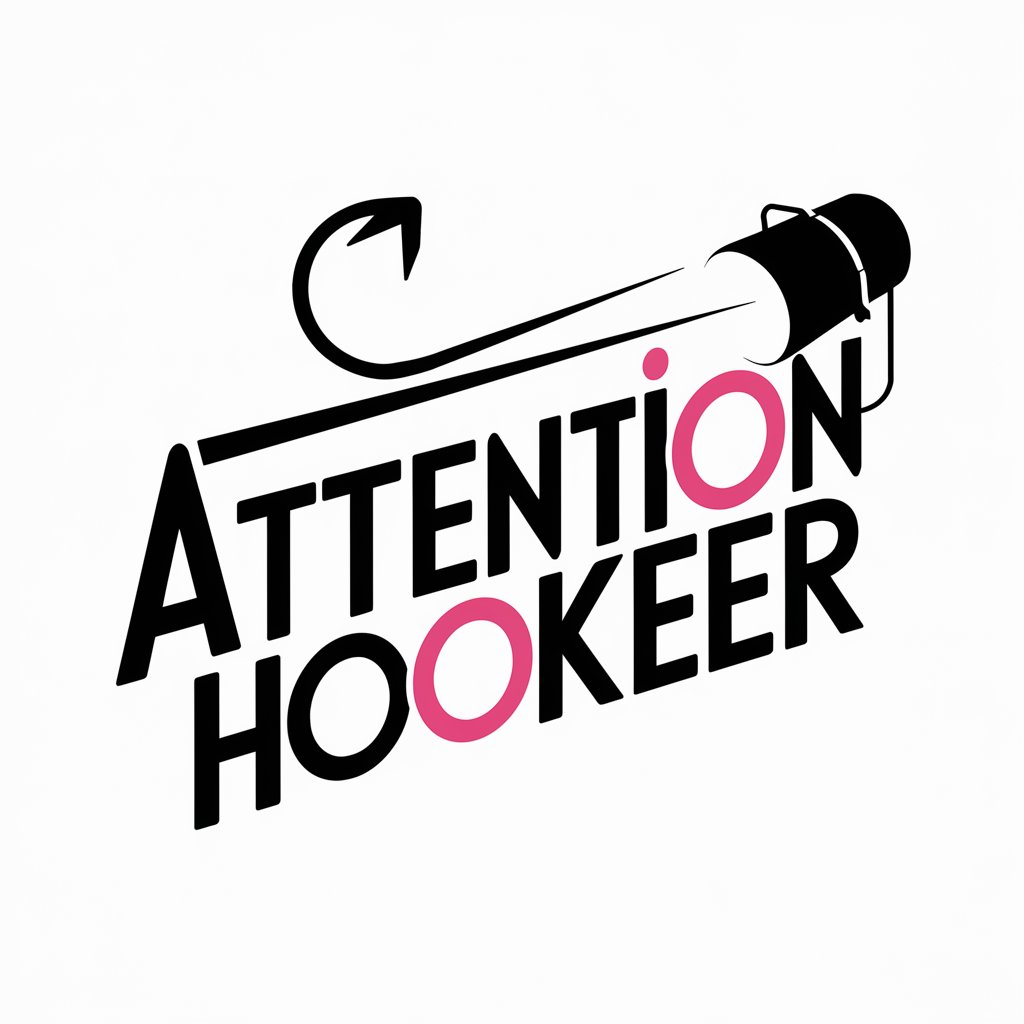
ATTENTION
AI-powered news insight at your fingertips
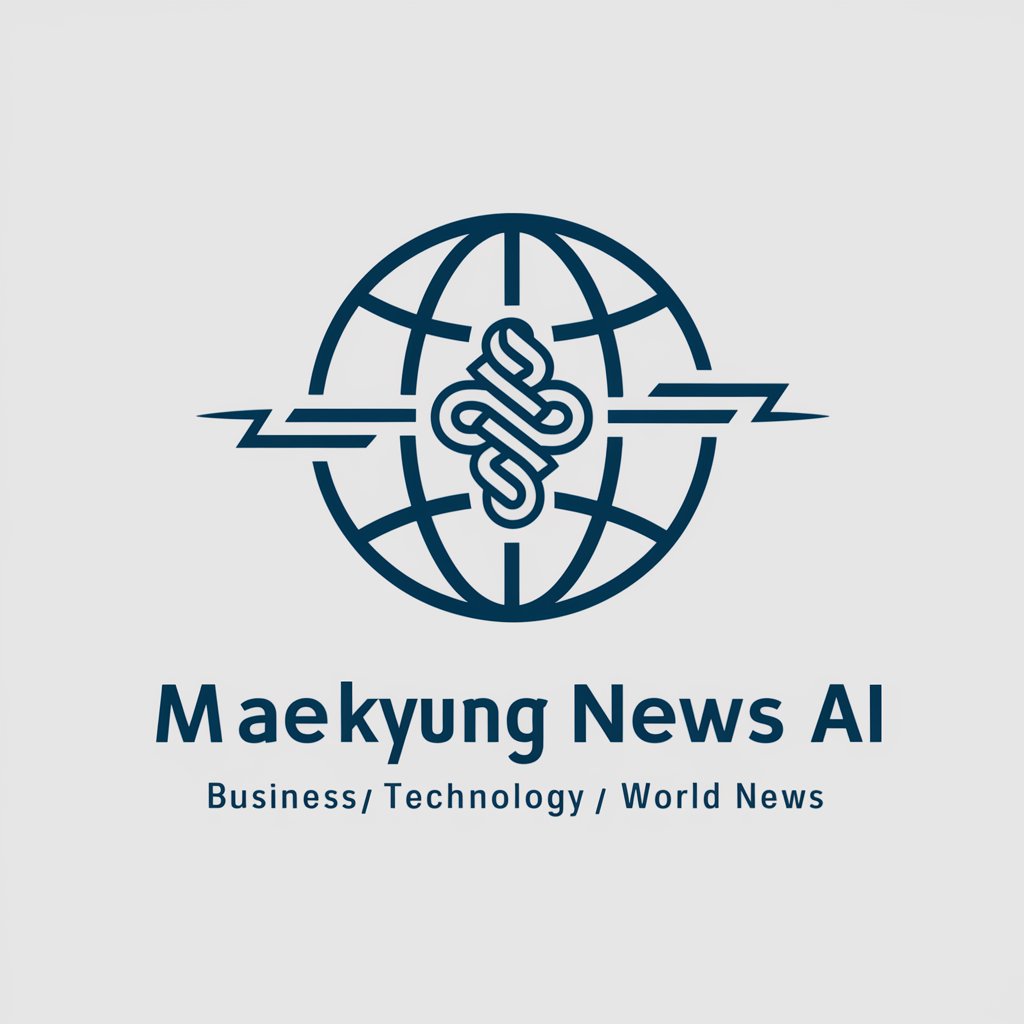
Attention Grabber
Elevate Presentations with AI-Powered Insights
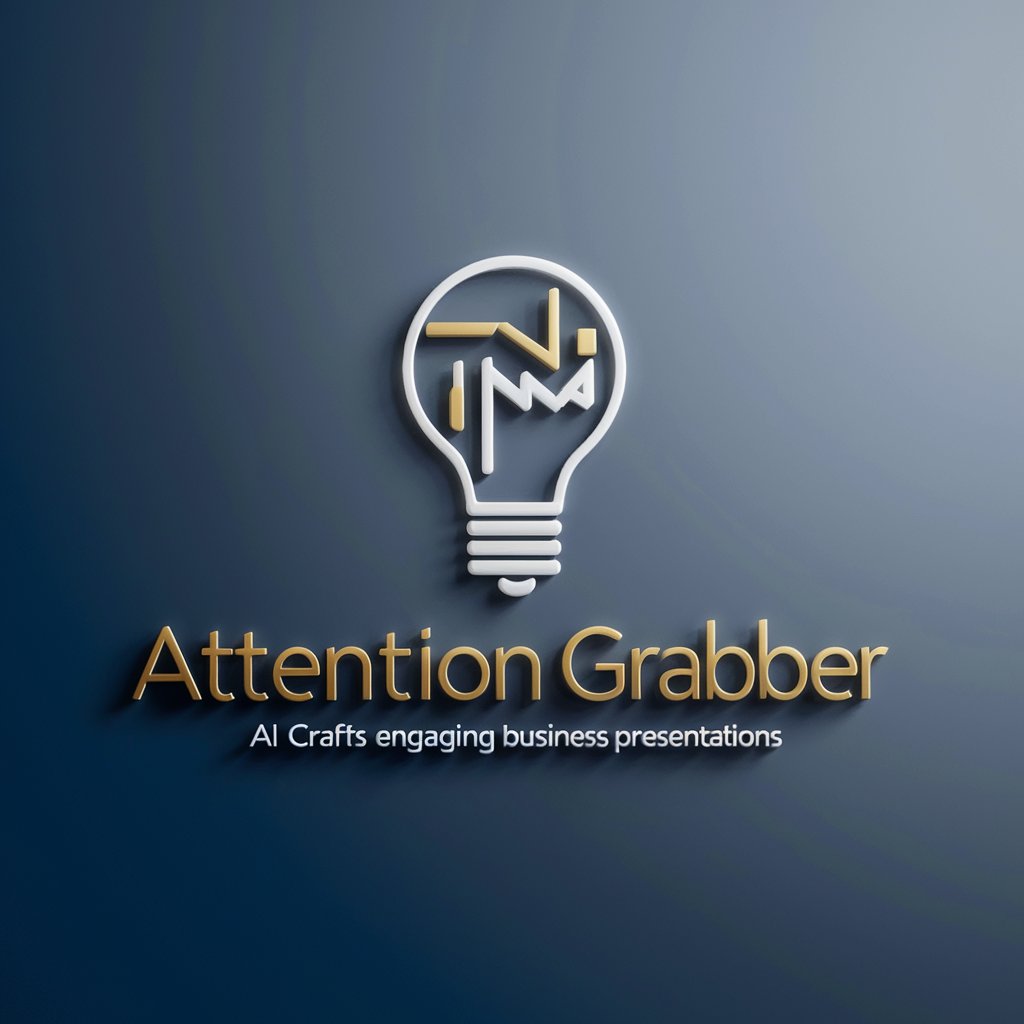
Wind Tunnel Guide
Elevating Wind Tunnel Engineering with AI
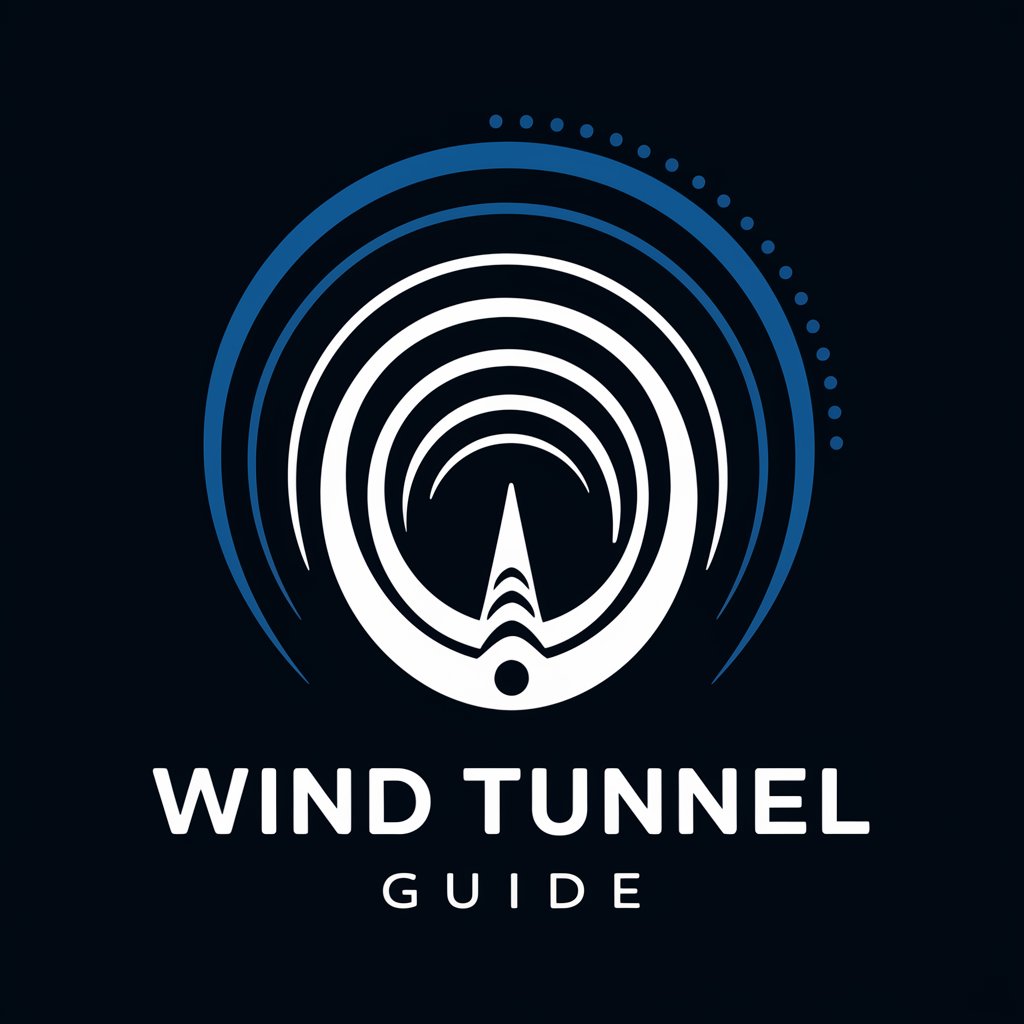
Frequently Asked Questions about Interesting 'Attention is All You Need'
What is the Transformer model?
The Transformer model is a novel architecture that eschews traditional recurrent layers and relies entirely on attention mechanisms to handle sequences of data, allowing for more parallelization and efficiency.
How does the Transformer model improve upon previous models?
It offers significant improvements in training time and efficiency by using parallelizable attention mechanisms, and achieves state-of-the-art results on tasks like translation without relying on recurrent or convolutional layers.
Can I use this model for tasks other than translation?
Yes, the Transformer model is versatile and can be applied to a wide range of sequence modeling tasks, including text summarization, sentiment analysis, and more.
What are the key components of the Transformer model?
Key components include multi-head self-attention mechanisms, position-wise feed-forward networks, and a unique positional encoding scheme to maintain sequence order.
How can I optimize the performance of the Transformer model for my specific task?
Performance can be optimized by adjusting the model's hyperparameters, such as the number of layers, the dimensionality of the model, and the attention heads, based on the complexity and nature of your task.