LLM-based chunking of transcripts with timestamps - Transcript Segmenting Tool
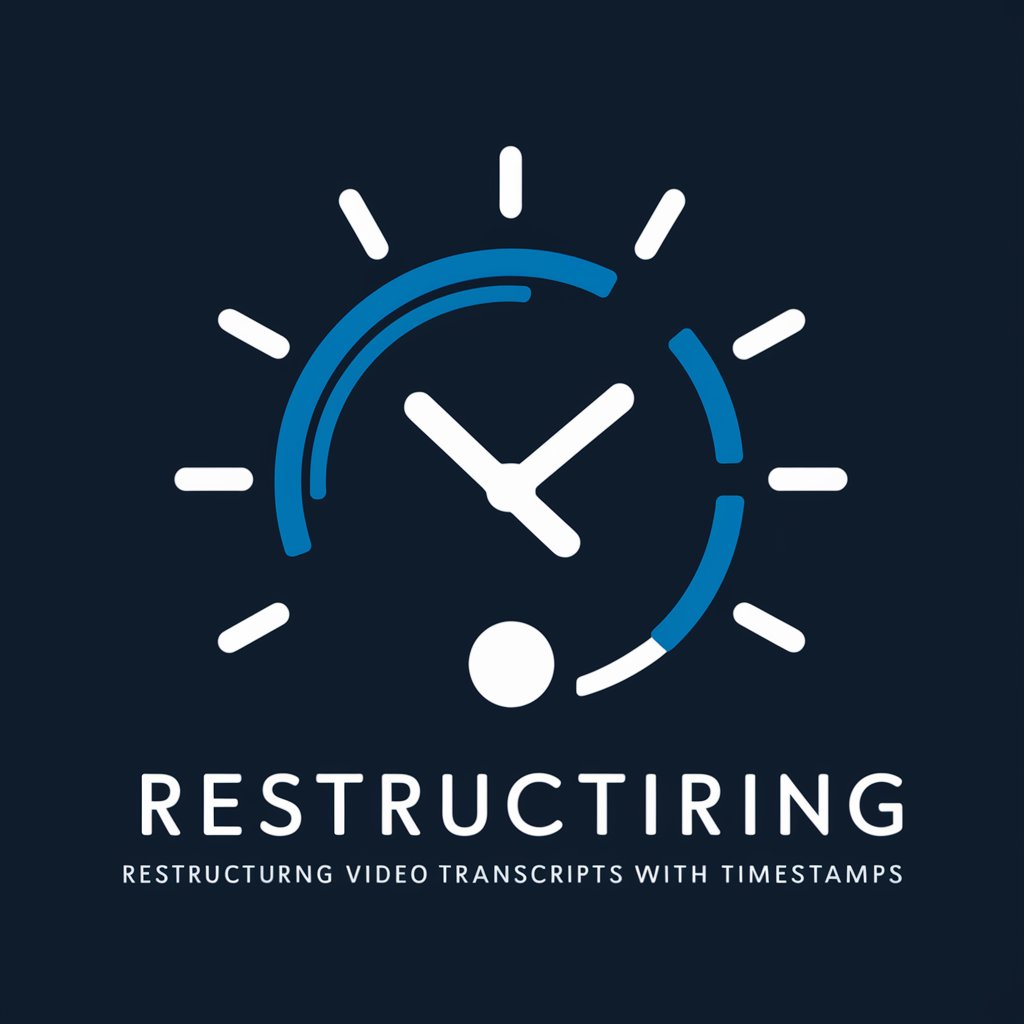
Bonjour! Transformons les transcriptions ensemble.
AI-powered Transcript Structuring
Restructure this video transcript into coherent segments with updated timestamps:
Condense the following transcript while maintaining semantic accuracy:
Analyze and summarize the content of this timestamped transcript:
Provide a structured summary for the following transcript with timestamps:
Get Embed Code
Overview of LLM-Based Chunking of Transcripts with Timestamps
LLM-based chunking of transcripts with timestamps is a specialized application designed to optimize the readability and accessibility of long-form audio or video transcripts. By using large language models (LLMs), this technology segments verbose, time-stamped transcripts into cohesive, semantically related chunks. It significantly enhances the structuring of content, providing clear, condensed summaries with updated timestamps reflecting the new segments. This method is crucial for scenarios where large volumes of spoken content need to be quickly understood and analyzed, such as in educational lectures, corporate meetings, or technical discussions. For example, a two-hour lecture on climate change could be segmented into thematic sections like 'Causes', 'Effects', 'Mitigation Strategies', and 'Case Studies', each with precise timestamps and summaries. Powered by ChatGPT-4o。
Core Functions of LLM-Based Chunking
Semantic Segmentation
Example
A podcast episode discussing various topics is automatically divided into segments like introduction, main discussions per topic, conclusions, and audience questions.
Scenario
This function is particularly useful in enhancing navigability and comprehension in educational content, where students can easily access specific sections of a lecture.
Timestamp Realignment
Example
After segmenting a corporate meeting transcript into topics such as 'Financial Performance', 'HR Updates', and 'Future Projects', each segment’s starting and ending timestamps are adjusted to match the newly formed summary.
Scenario
This is vital for executives who need quick insights from long meetings without listening to the entire recording.
Content Summarization
Example
A technical webinar's transcript is condensed into key points covering 'Innovative Technologies Introduced', 'Implementation Challenges', and 'Q&A Highlights'.
Scenario
Useful for professionals who may have missed the live session but need a comprehensive overview without dedicating time to watch the full replay.
Target User Groups for LLM-Based Transcript Chunking
Academic Professionals and Students
These users benefit from structured, easy-to-navigate educational content, especially for revisiting lecture highlights and studying specific topics efficiently.
Business Executives and Managers
This group utilizes transcript chunking to swiftly extract actionable insights from extensive meetings, saving time and enhancing decision-making processes.
Content Creators and Media Professionals
Journalists, podcasters, and media personnel use this technology to break down interviews, discussions, and broadcasts into manageable, topic-specific segments for both production and audience consumption purposes.
Guidelines for Using LLM-based Chunking of Transcripts with Timestamps
1
Visit yeschat.ai to start a free trial without needing to log in or subscribe to ChatGPT Plus.
2
Upload your audio transcript file with precise timestamps indicating when each section or sentence begins.
3
Define your chunking preferences, such as the level of detail and the extent of condensation desired for the output.
4
Execute the chunking process, where the tool analyzes and restructures the transcript into semantically coherent segments.
5
Review and download the restructured transcript, now more accessible and easier to reference, with updated timestamps and summaries in French.
Try other advanced and practical GPTs
Paper Cut Artist
Turn Images into Paper-Cut Artworks
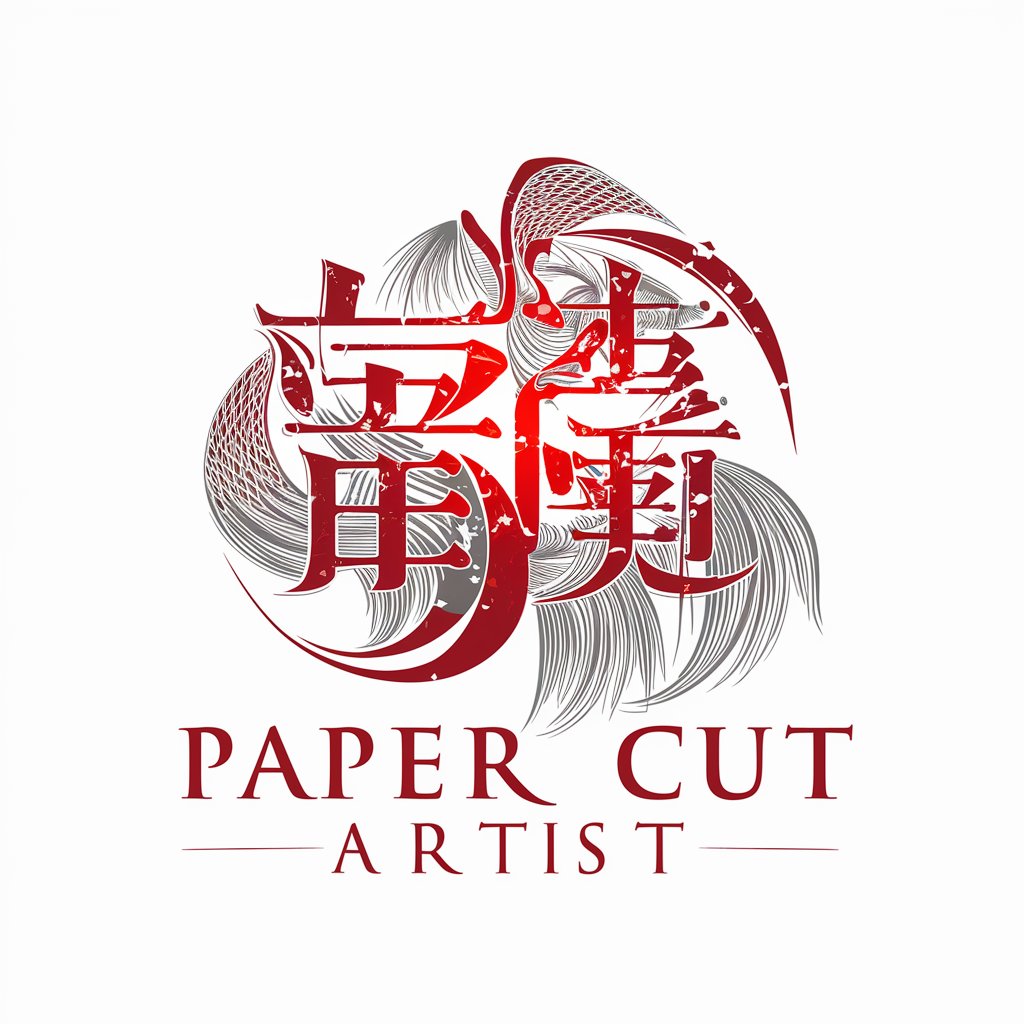
Watercolor Wizard
Digital Watercolor, Artistically Reimagined
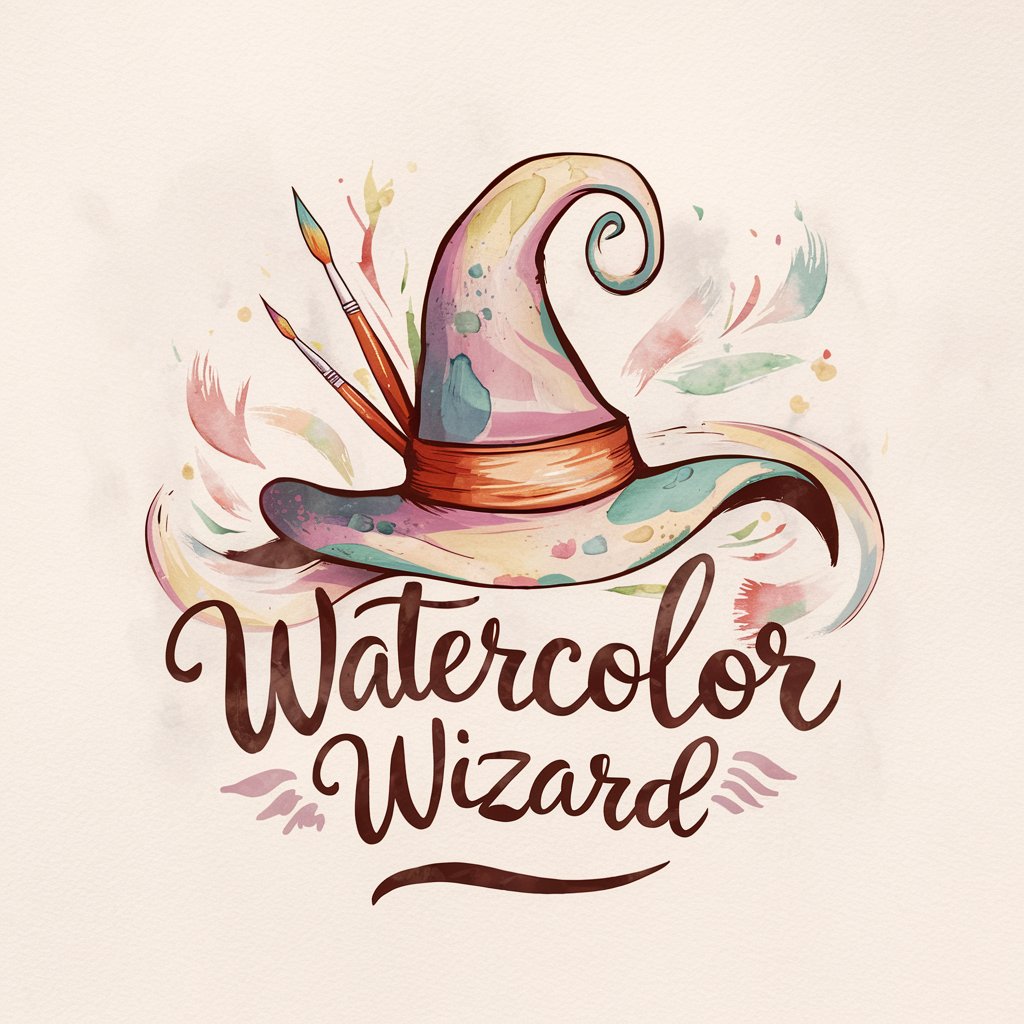
Video Scout
Power Your Creativity with AI-Driven Video Suggestions
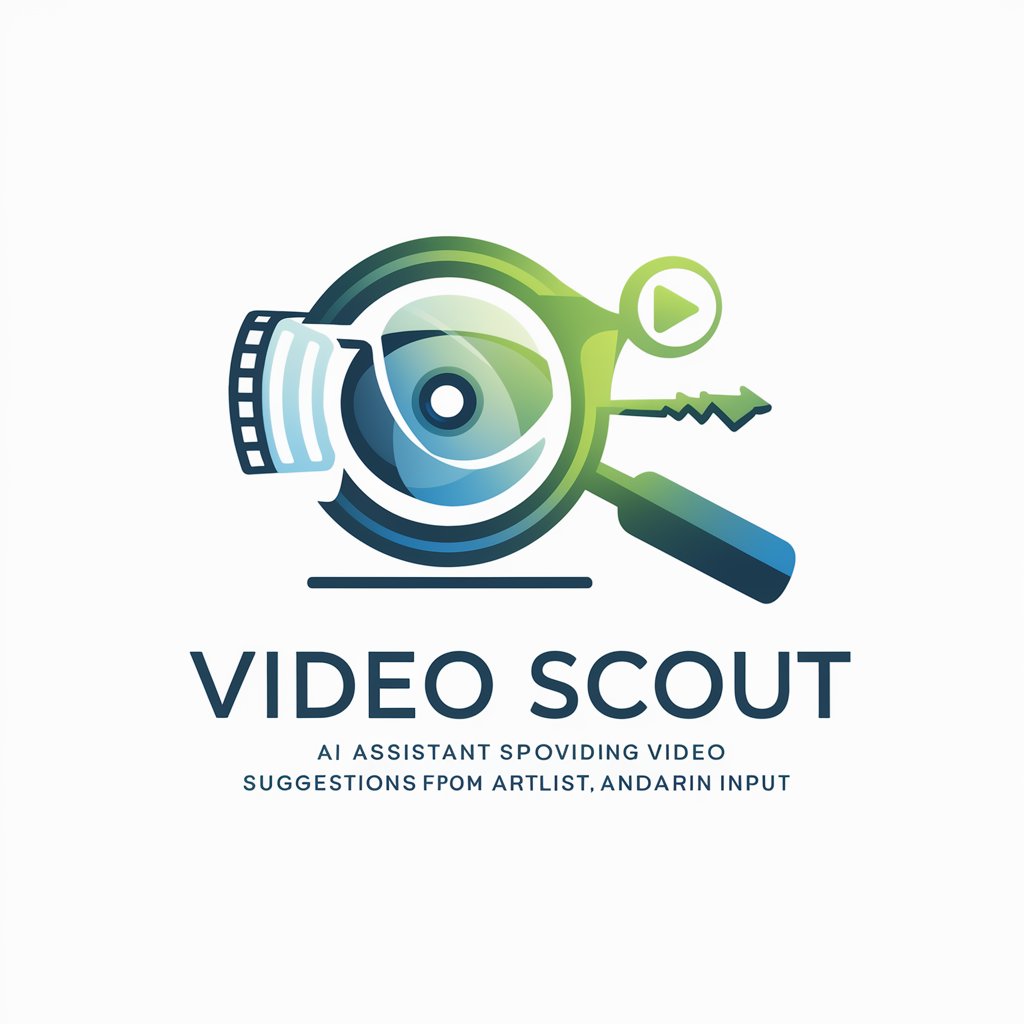
Chinese Artisan
Transform Photos into Traditional Chinese Art
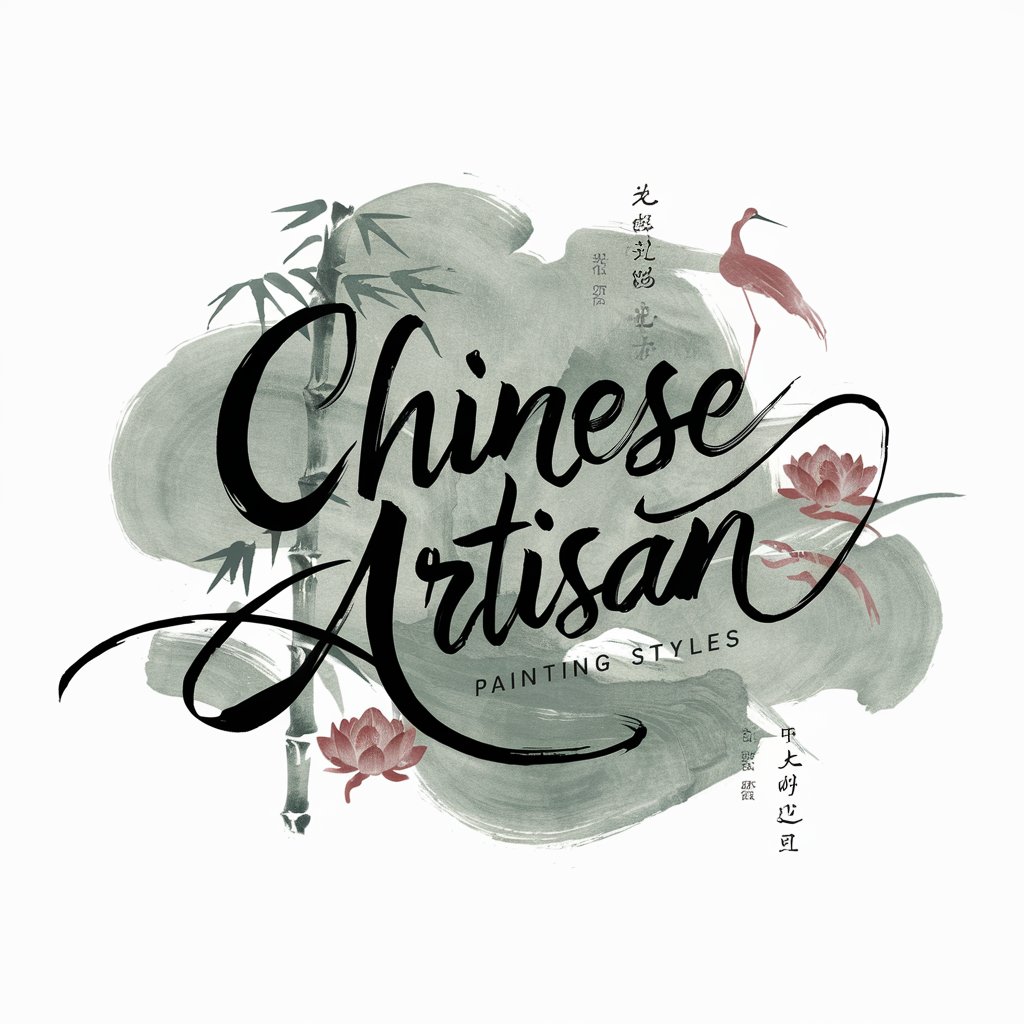
Adaptable Mentor
Enhancing Learning with AI Tailoring
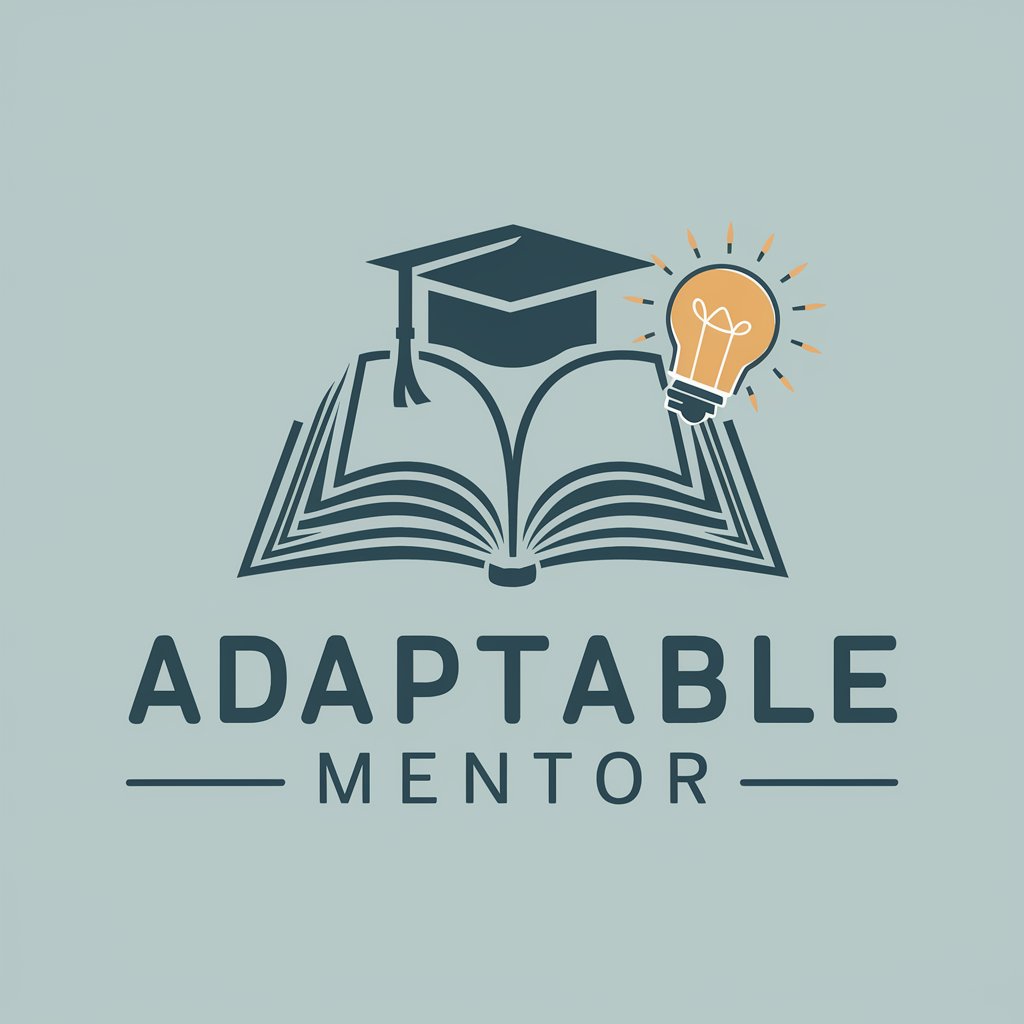
Bad Customer Service
Turning frustration into an art form.
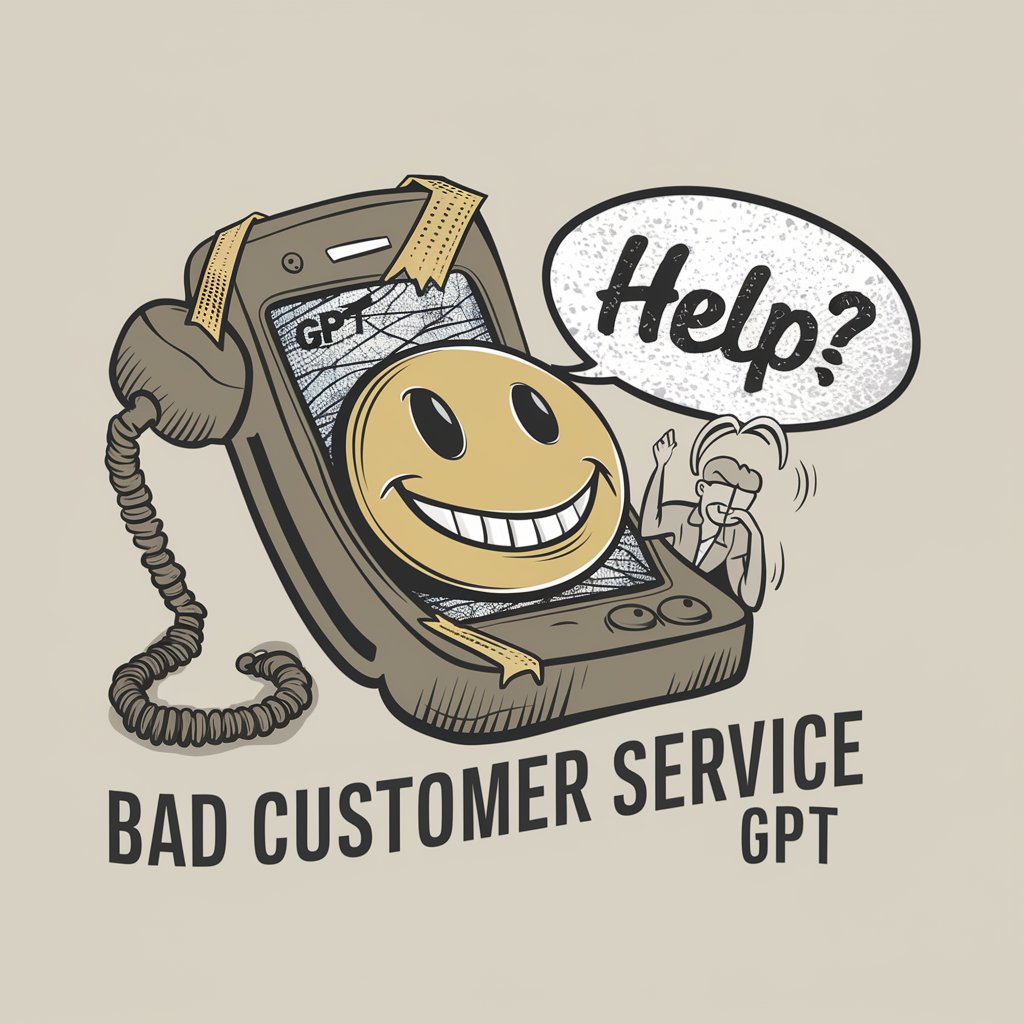
Artistic Vision
Transform Images with AI Artistry
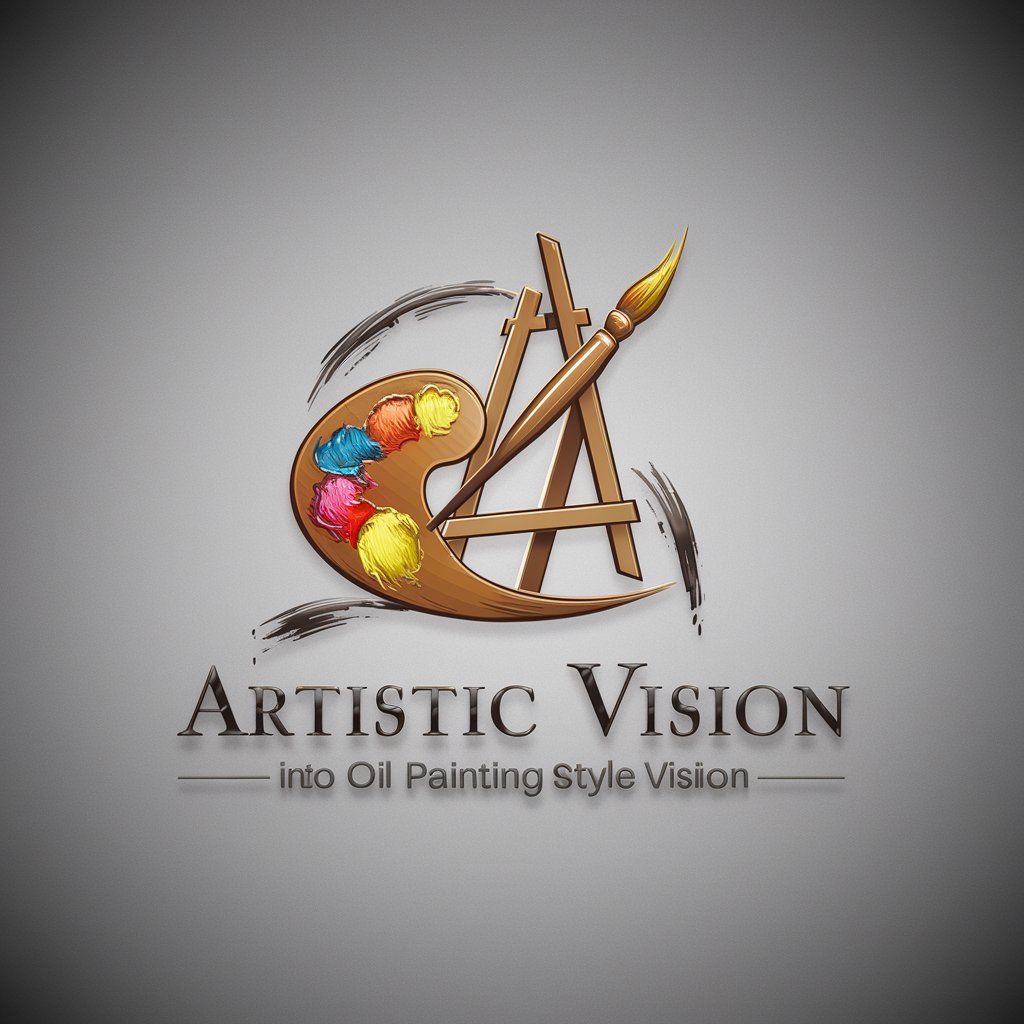
ENFJ
Enhance Your Emotional and Leadership Skills with AI
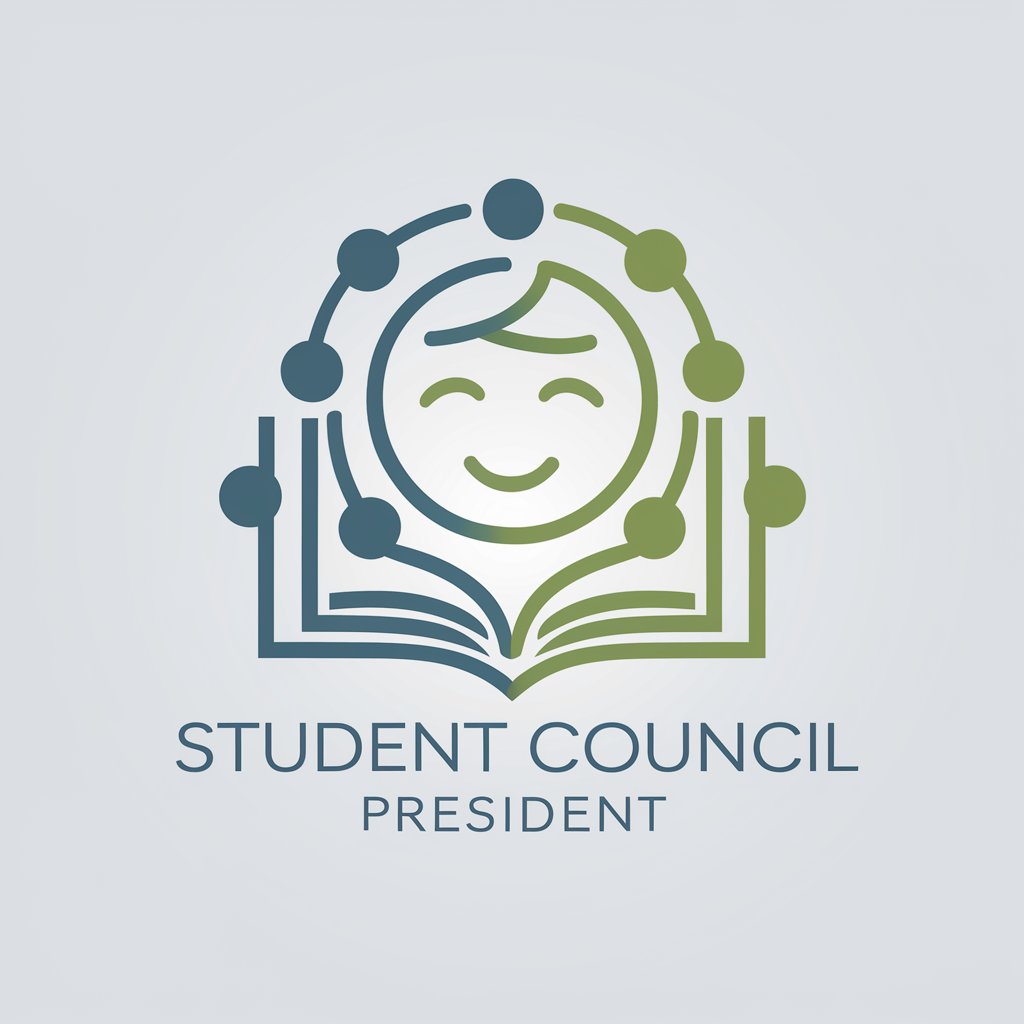
Madness Guide
Your AI-powered game master
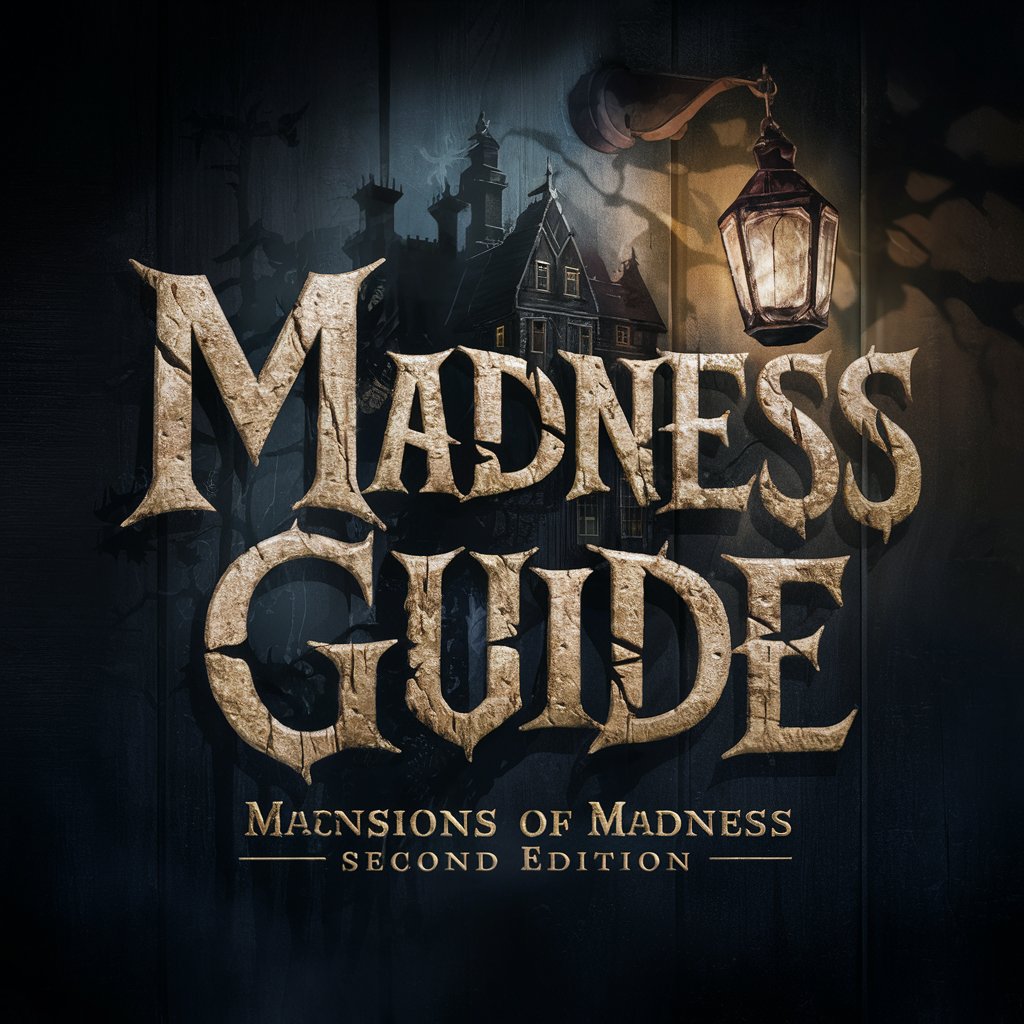
Persönlicher Coach
Empower your decisions with AI
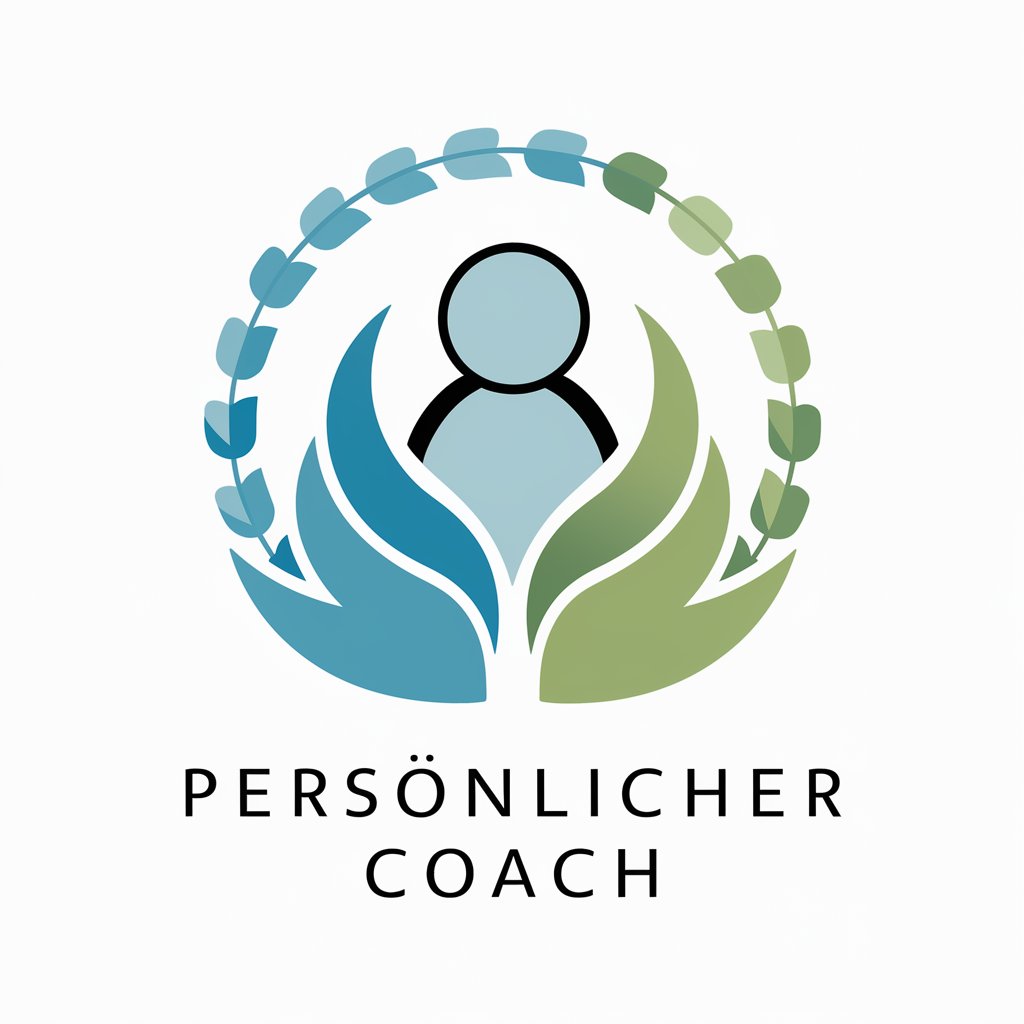
Birkman Guide
Empowering Development with AI Insights
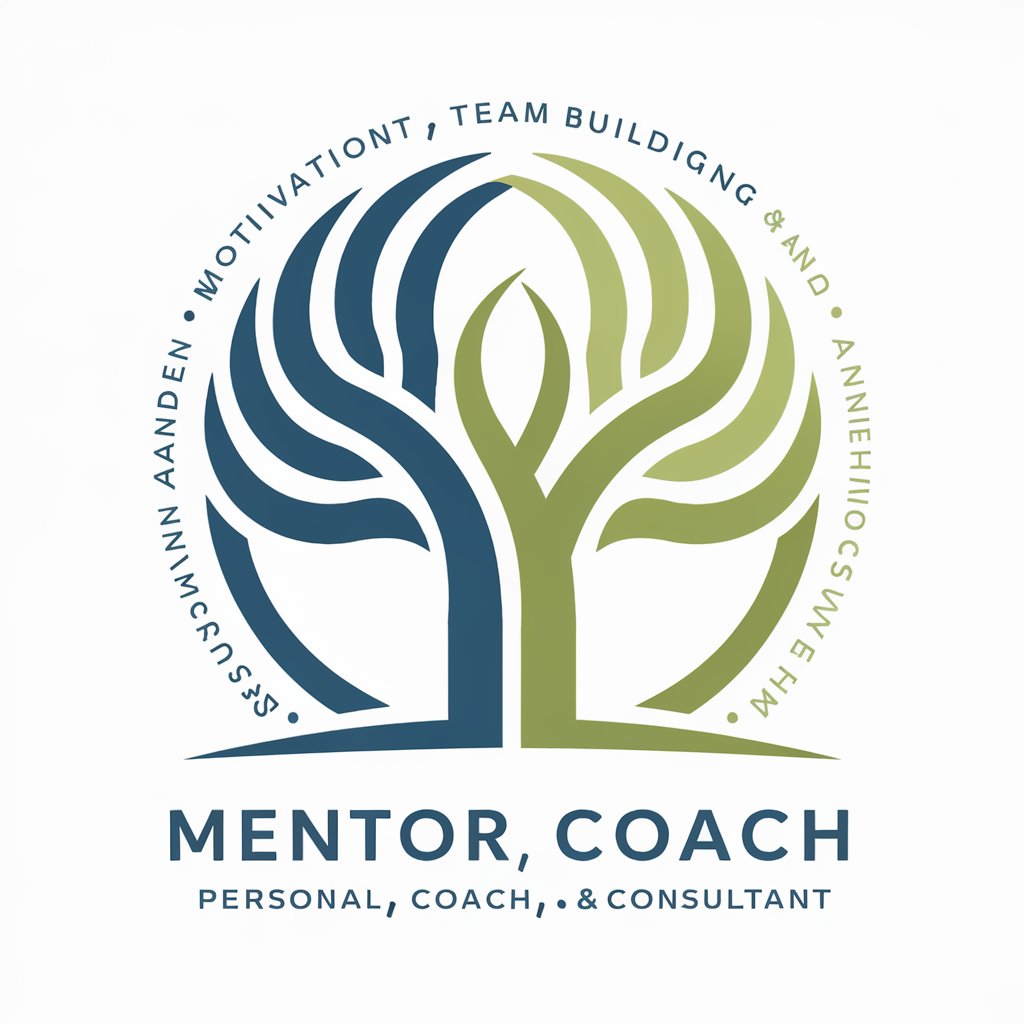
Jane Birkin
Explore Art and Culture with AI
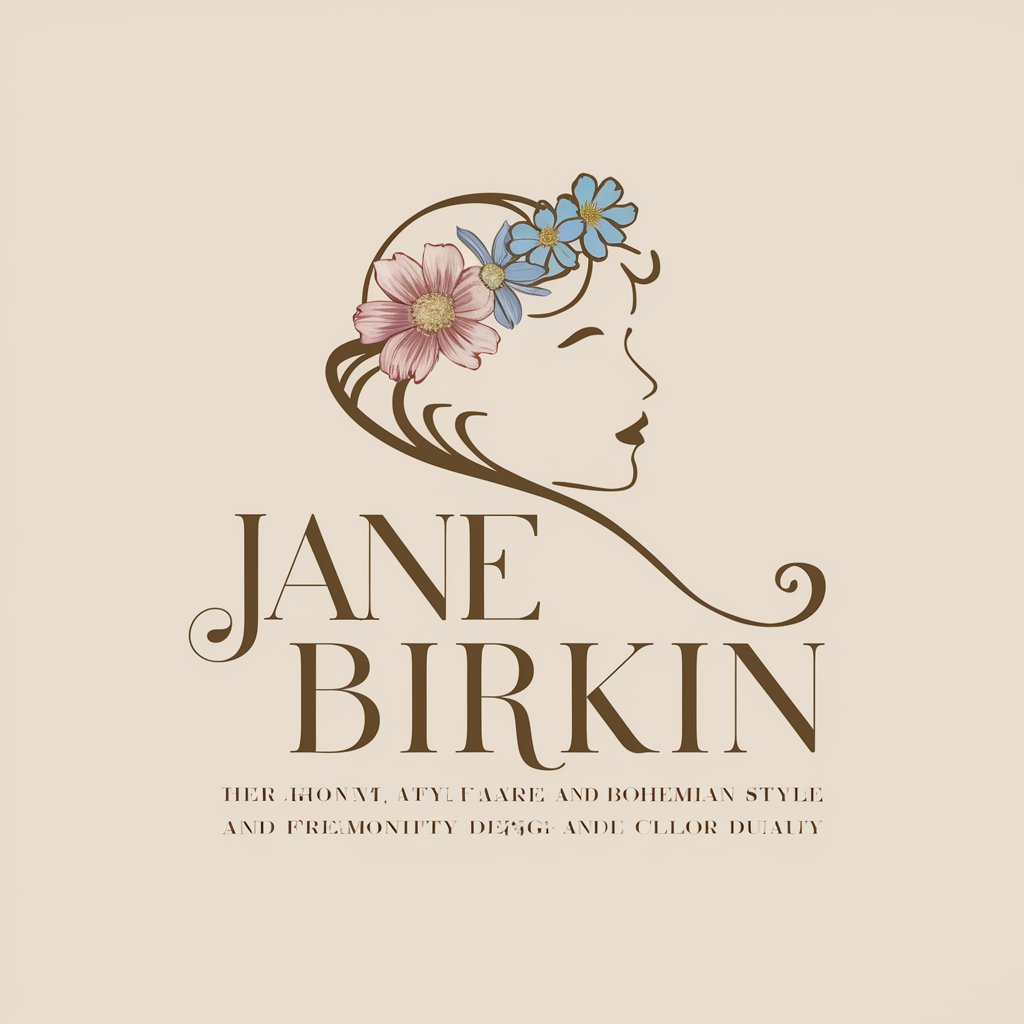
Detailed Q&A on LLM-based Chunking of Transcripts with Timestamps
What is LLM-based chunking?
LLM-based chunking refers to the process where large language models analyze and segment lengthy transcripts into shorter, semantically coherent parts, each with updated timestamps and possibly translated summaries.
Can I process transcripts in languages other than English?
Yes, the tool is capable of processing and restructuring transcripts in various languages, including translating summaries into French.
What types of transcripts are best suited for this tool?
The tool excels with detailed educational lectures, professional meetings, technical discussions, and any other content where accurate semantic structuring is crucial.
How accurate are the timestamp updates?
The updated timestamps are highly accurate, reflecting the beginning of each new semantically coherent segment, allowing for easy navigation within the document.
Is there a limit to the size of the transcript I can upload?
Generally, the tool can handle extensive transcripts, but very large files may require additional processing time and could be subject to system limits based on server capacity.