7 GPTs for Synthesis Planning Powered by AI for Free of 2024
AI GPTs for Synthesis Planning refer to advanced generative pre-trained transformer models specifically developed or adapted to assist in the planning and execution of synthetic processes, such as chemical synthesis or material fabrication. These AI tools leverage the power of machine learning and natural language processing to understand complex instructions, analyze research data, and suggest optimal pathways for synthesis, making them invaluable in fields like chemistry, pharmacology, and materials science. Their role is to streamline the synthesis planning process, reduce experimental trial and error, and enhance innovation by providing data-driven insights and recommendations.
Top 6 GPTs for Synthesis Planning are: The Chemist,Organic Synthesis Chem Lab,Organic Chemistry Synthesis Assistant,Pro Chemistry Lab Assistant,Organic Chemistry,🧪 ChemConnect Interactor 🌐
The Chemist
AI-Powered Organic Chemistry Mastery
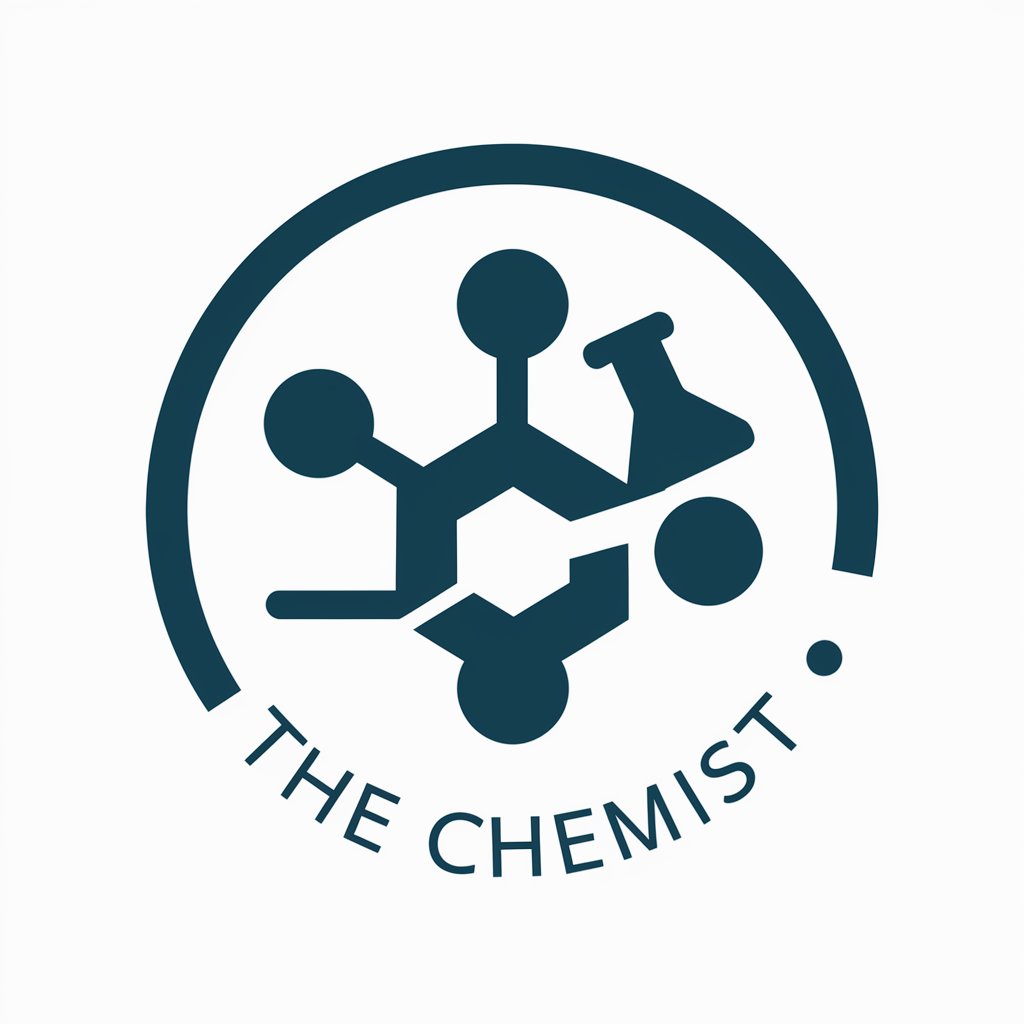
Organic Synthesis Chem Lab
Revolutionizing molecule synthesis with AI
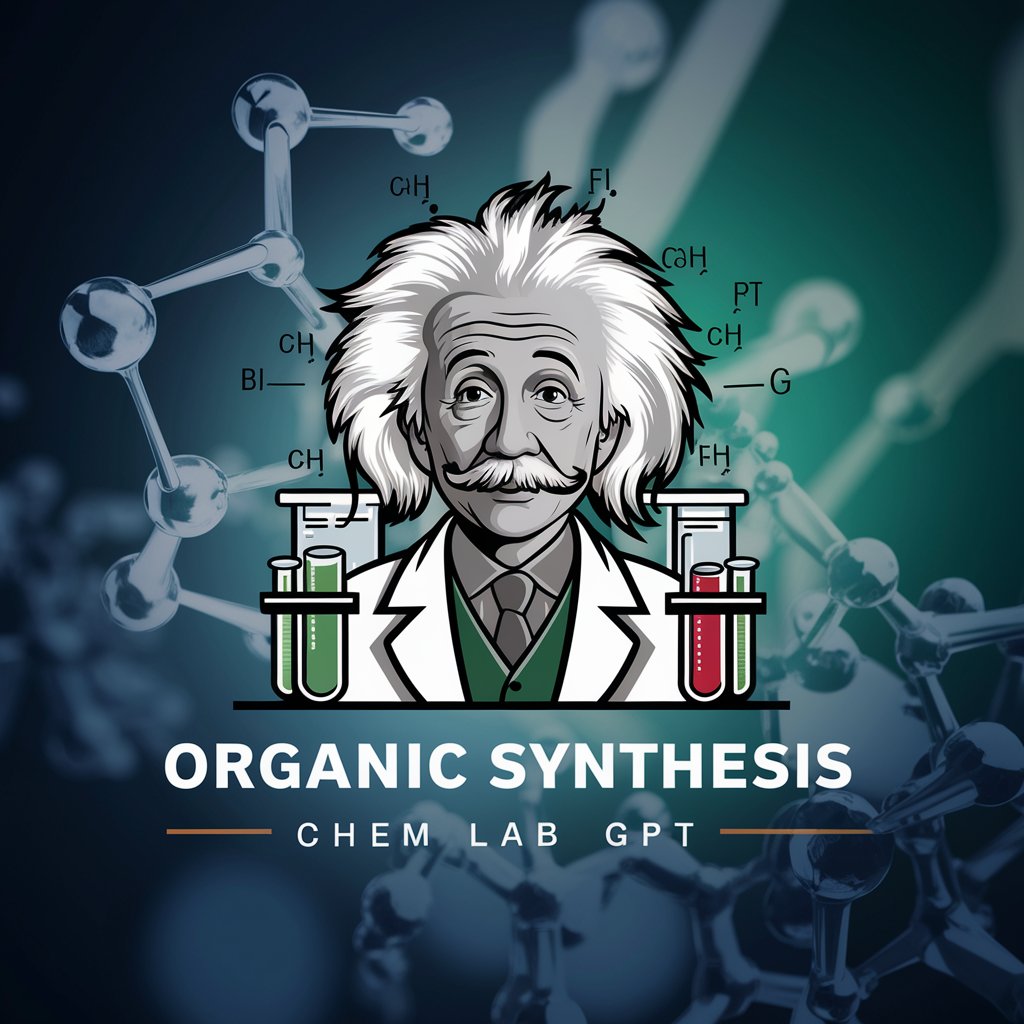
Organic Chemistry Synthesis Assistant
AI-driven organic synthesis insights
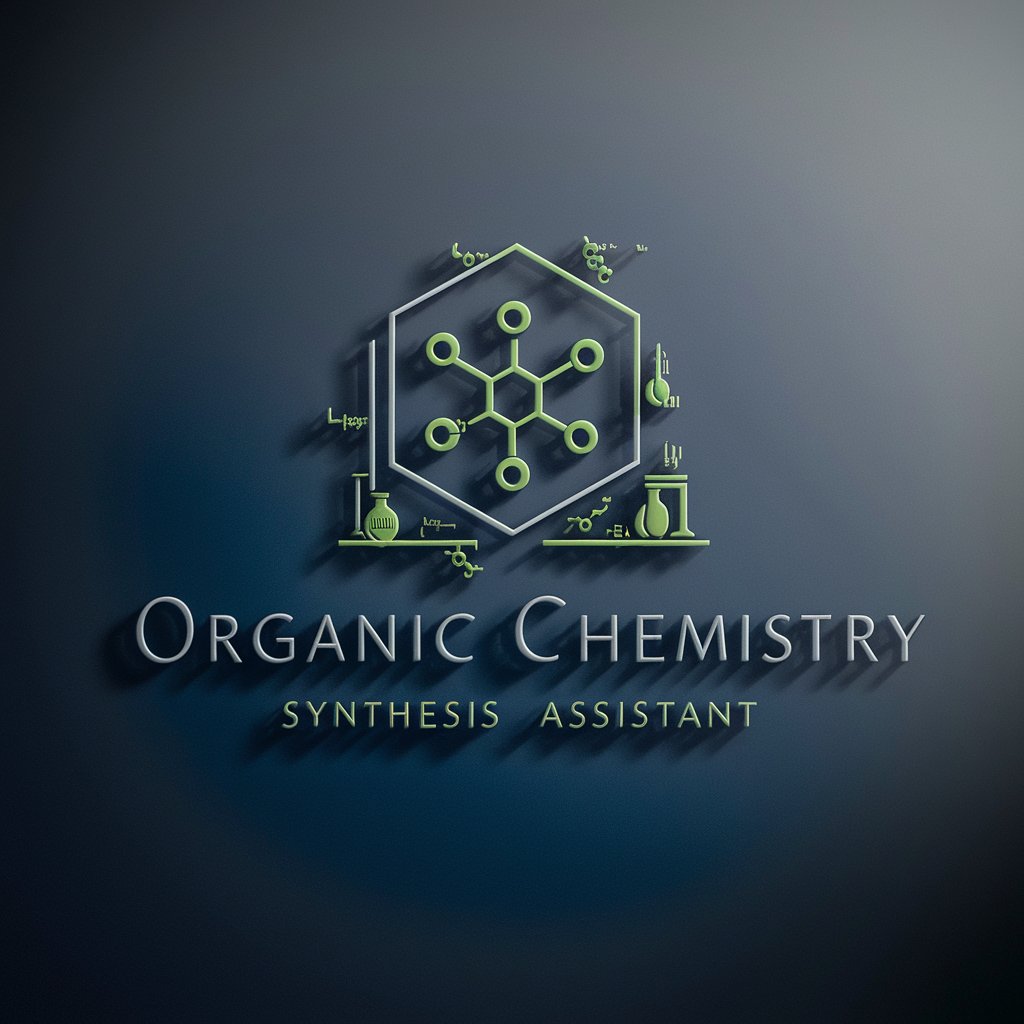
Pro Chemistry Lab Assistant
Empowering Chemistry with AI
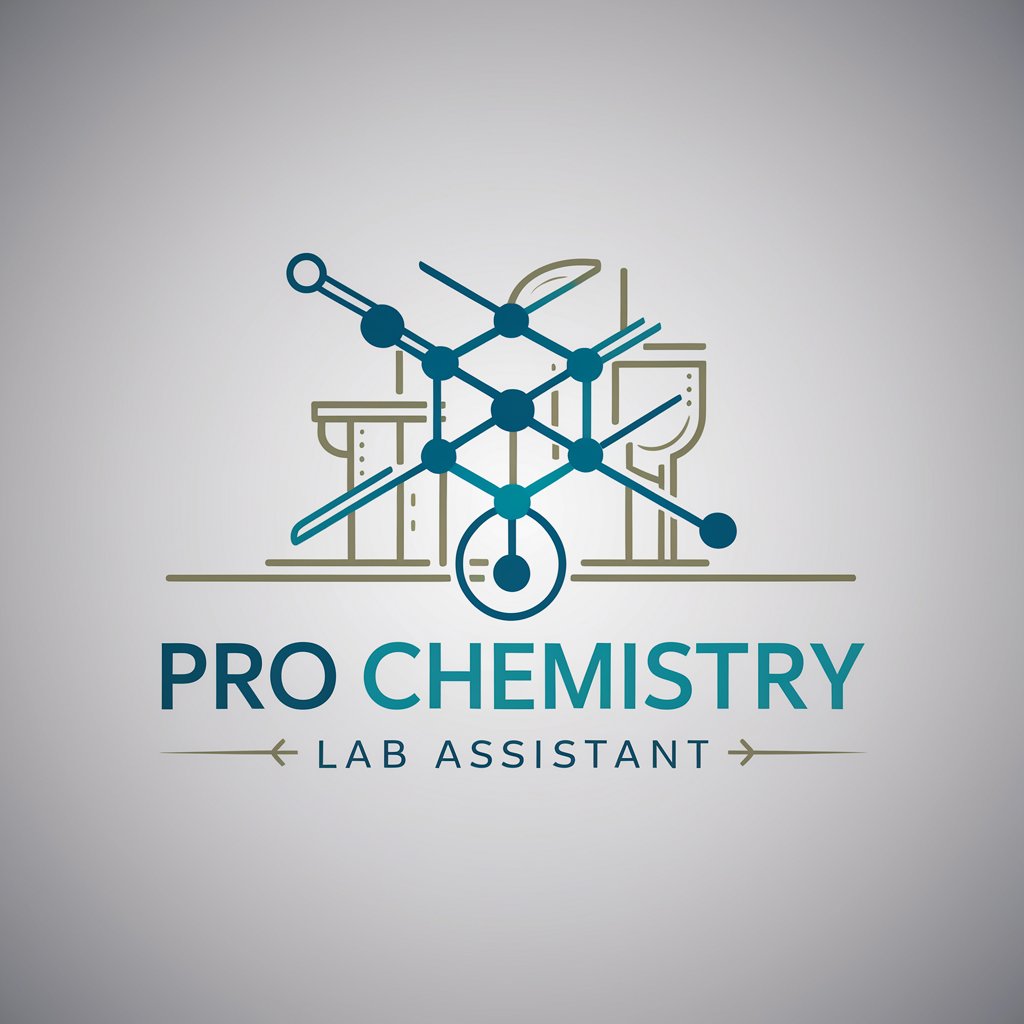
Organic Chemistry
Decoding Organic Chemistry with AI
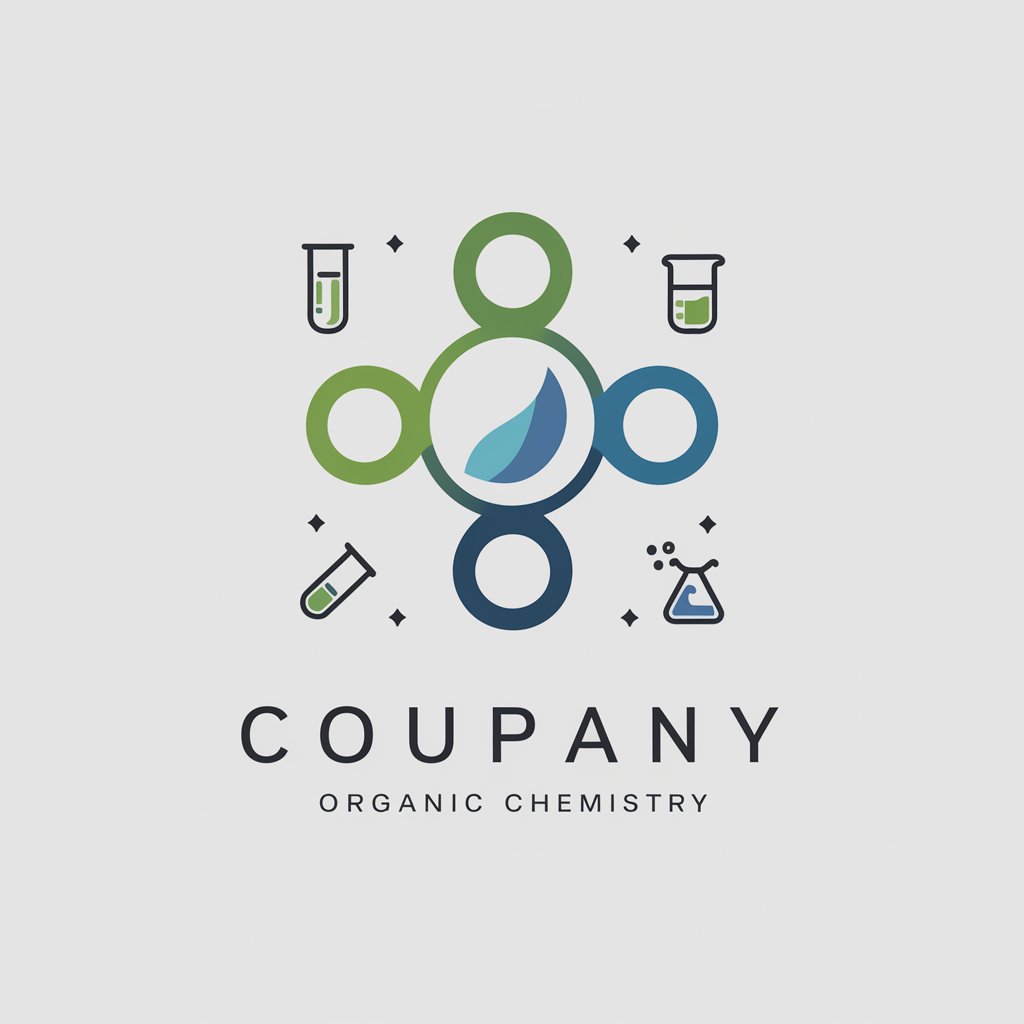
🧪 ChemConnect Interactor 🌐
Predicting Chemistry with AI

Key Characteristics and Capabilities
AI GPTs tailored for Synthesis Planning boast a range of unique features that cater to the intricacies of synthesis processes. These include advanced language comprehension for parsing scientific literature, data analysis tools for interpreting experimental results, and predictive modeling to forecast synthesis outcomes. They are adaptable, scaling from straightforward substance combinations to intricate molecular designs. Special features may encompass real-time web searching for the latest studies, image generation for visualizing molecular structures, and custom code execution for specialized data analysis, enhancing their utility in synthesis planning.
Who Benefits from Synthesis Planning AI
The primary users of AI GPTs for Synthesis Planning span from beginners in the field, such as students learning about synthesis, to experts, including chemists, material scientists, and pharmacologists. These tools are designed to be user-friendly for those without programming knowledge, offering intuitive interfaces and guided workflows. Simultaneously, they provide advanced customization and programmable features for developers and professionals with technical expertise, allowing for tailored solutions to complex synthesis challenges.
Try Our other AI GPTs tools for Free
Feedback Solicitation
Discover how AI GPTs for Feedback Solicitation transform feedback collection with advanced analysis, making it easier for businesses to gather actionable insights.
Story Generation
Explore the world of AI GPTs for Story Generation, your ultimate tool for creating captivating narratives with ease. Perfect for writers, educators, and creatives seeking innovative storytelling solutions.
Imaginative Roleplay
Explore the world of AI GPTs for Imaginative Roleplay: versatile AI tools designed to enhance storytelling, character development, and interactive narratives for creators at all levels.
Assertiveness Training
Discover how AI-powered GPTs transform assertiveness training with personalized, interactive learning experiences. Enhance your communication skills confidently and effectively.
Charismatic Communication
Explore the future of engagement with AI GPTs for Charismatic Communication. Designed to enhance interactions, these tools offer tailored, persuasive messaging for anyone looking to elevate their communication.
Art Identification
Discover the transformative power of AI GPTs in Art Identification, your essential tool for exploring, categorizing, and understanding art in all its forms.
Further Observations on Customized GPT Solutions
AI GPTs for Synthesis Planning exemplify the customization potential of AI across different sectors, offering user-friendly solutions that adapt to specific industry needs. These tools not only enhance efficiency and innovation in synthesis planning but also pave the way for their integration into broader scientific research and development workflows, promising significant advancements in how research is conducted and applied in real-world applications.
Frequently Asked Questions
What exactly are AI GPTs for Synthesis Planning?
AI GPTs for Synthesis Planning are specialized AI models that assist in designing and optimizing synthetic processes, leveraging machine learning to analyze data and predict effective synthesis pathways.
How can these AI tools benefit synthesis planning?
They streamline the planning process, reduce the need for extensive experimental trials, and offer insights based on a vast array of scientific data, thereby accelerating research and development.
Do I need programming skills to use these AI GPTs?
No, these tools are designed to be accessible for users without programming skills, featuring user-friendly interfaces and guided operations.
Can experts customize these AI tools for specific needs?
Yes, they offer advanced customization options, including programmable features and APIs, to cater to specific research requirements and complex synthesis tasks.
Are these tools capable of processing scientific literature?
Absolutely, they are equipped with language comprehension capabilities to parse and analyze scientific texts, extracting relevant data for synthesis planning.
Can AI GPTs predict the outcomes of synthesis processes?
Yes, through predictive modeling, these AI tools can forecast possible outcomes, helping researchers to optimize synthesis routes and conditions.
How do these tools integrate with existing research workflows?
They are designed to complement existing workflows by providing data analysis, literature review, and predictive insights, seamlessly integrating with traditional research methods.
What advancements are expected in the future for these AI tools?
Future developments include enhanced predictive accuracy, broader data integration, and more intuitive interfaces, further simplifying synthesis planning and expanding their applicability across various fields.