Latent Consistency Model - Efficient Image Synthesis
Hello
Empowering Creativity with AI
Get Embed Code
Introduction to Latent Consistency Models
Latent Consistency Models (LCMs) are an innovative approach to synthesizing high-resolution images with few-step inference, built on the foundation of latent diffusion models (LDMs). The core objective of LCMs is to address the computational intensity and slow generation speed of traditional iterative sampling processes in LDMs by enabling rapid, high-fidelity sampling in the latent space of pre-trained models such as Stable Diffusion. By viewing the guided reverse diffusion process as solving an augmented probability flow ODE (PF-ODE), LCMs are designed to directly predict the solution of such ODE in latent space, thus significantly reducing the need for numerous iterations. This direct approach allows for swift generation of high-quality images with minimal steps, leveraging the underlying structure of pre-existing models without the need for extensive retraining. For example, an LCM can distill a pre-trained Stable Diffusion model into a format that can generate 768×768 resolution images in 2∼4 steps or even one step, a process that originally might require thousands of iterations. Powered by ChatGPT-4o。
Main Functions of Latent Consistency Models
Swift High-Resolution Image Generation
Example
Generating 768×768 resolution images from textual descriptions in just 2-4 steps.
Scenario
In a scenario where a digital artist needs to quickly generate high-resolution concept art based on textual descriptions, LCMs can produce quality images rapidly, allowing for immediate feedback and iteration on creative projects.
Few-Step Inference on Customized Datasets
Example
Fine-tuning LCMs for specific image styles or domains with minimal computational resources.
Scenario
For a small video game development studio looking to generate unique character and environment art, LCMs allow for the fine-tuning of models on their proprietary datasets, ensuring the generated images adhere to their specific aesthetic needs without the overhead of training a model from scratch.
Efficient Distillation from Pre-trained Models
Example
Distilling Stable Diffusion into a 2∼4-step LCM.
Scenario
AI research teams can leverage LCMs to distill complex, pre-trained diffusion models into more efficient versions that maintain high fidelity while dramatically reducing inference time, facilitating more practical applications of generative models in real-time systems.
Ideal Users of Latent Consistency Model Services
Digital Artists and Creative Professionals
Individuals seeking to rapidly prototype and visualize ideas can use LCMs to generate high-quality images from textual descriptions, streamlining the creative process and enabling faster iteration on visual concepts.
AI Researchers and Developers
Professionals working on the cutting edge of AI and machine learning can utilize LCMs to explore new methods of model efficiency, distillation, and the generation of synthetic data for various applications, from training datasets to content creation.
Small to Medium Enterprises (SMEs) in Content Creation
Companies in the gaming, animation, and digital marketing sectors can benefit from LCMs by generating custom content that fits their unique brand identity or project requirements without the significant costs associated with traditional content production methods.
How to Use Latent Consistency Model
Step 1
Start your journey at yeschat.ai to explore Latent Consistency Model with a free trial, no sign-in or ChatGPT Plus subscription required.
Step 2
Ensure you have a basic understanding of machine learning concepts, especially diffusion models and latent space, to effectively apply the model to your tasks.
Step 3
Choose your application area, such as text-to-image generation or dataset fine-tuning, to focus the model's capabilities on your specific needs.
Step 4
Integrate Latent Consistency Model into your workflow by using the provided API or library functions, adhering to the documentation for technical guidelines.
Step 5
Experiment with different configurations and parameters to optimize the model's performance for your unique application, utilizing community forums or support for advice and best practices.
Try other advanced and practical GPTs
TidyMate
Declutter your space with AI-powered guidance
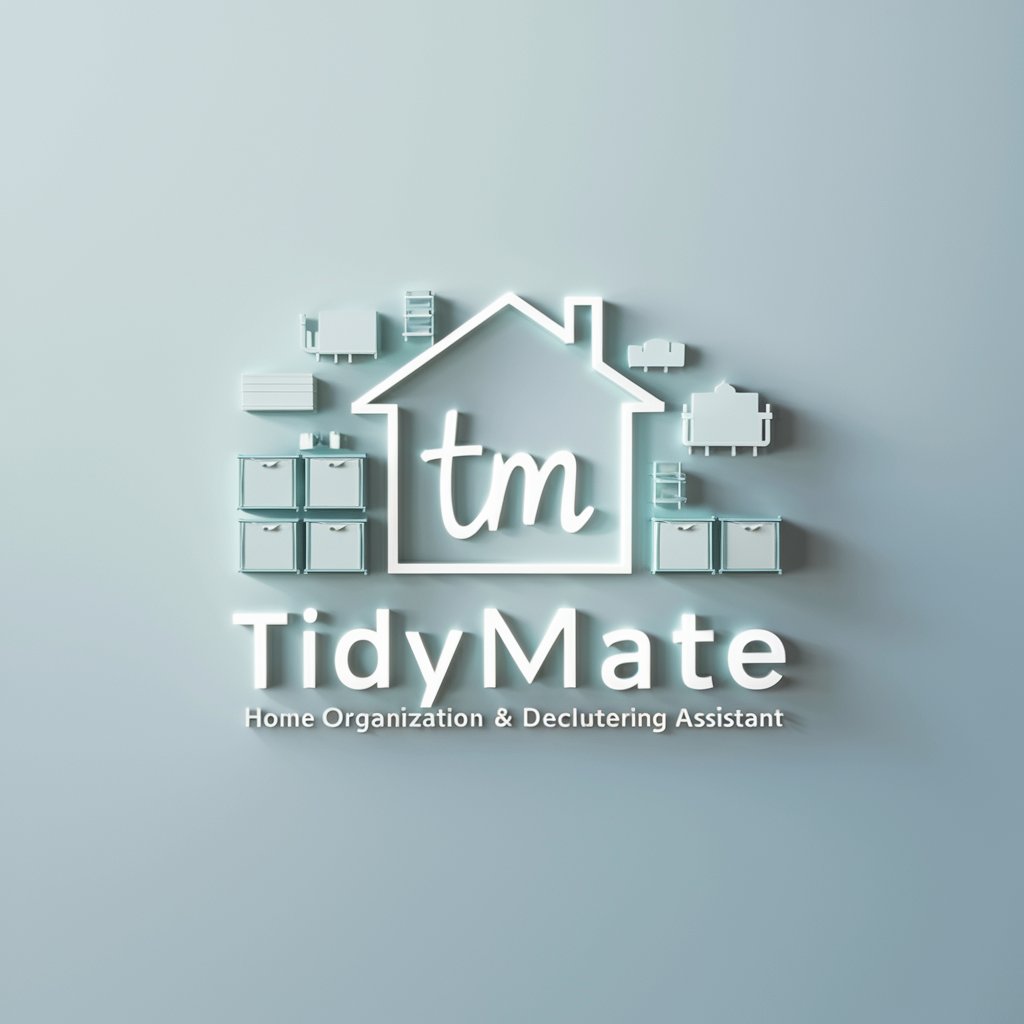
Kemi - Research & Creative Assistant
Empowering Creativity and Insight with AI
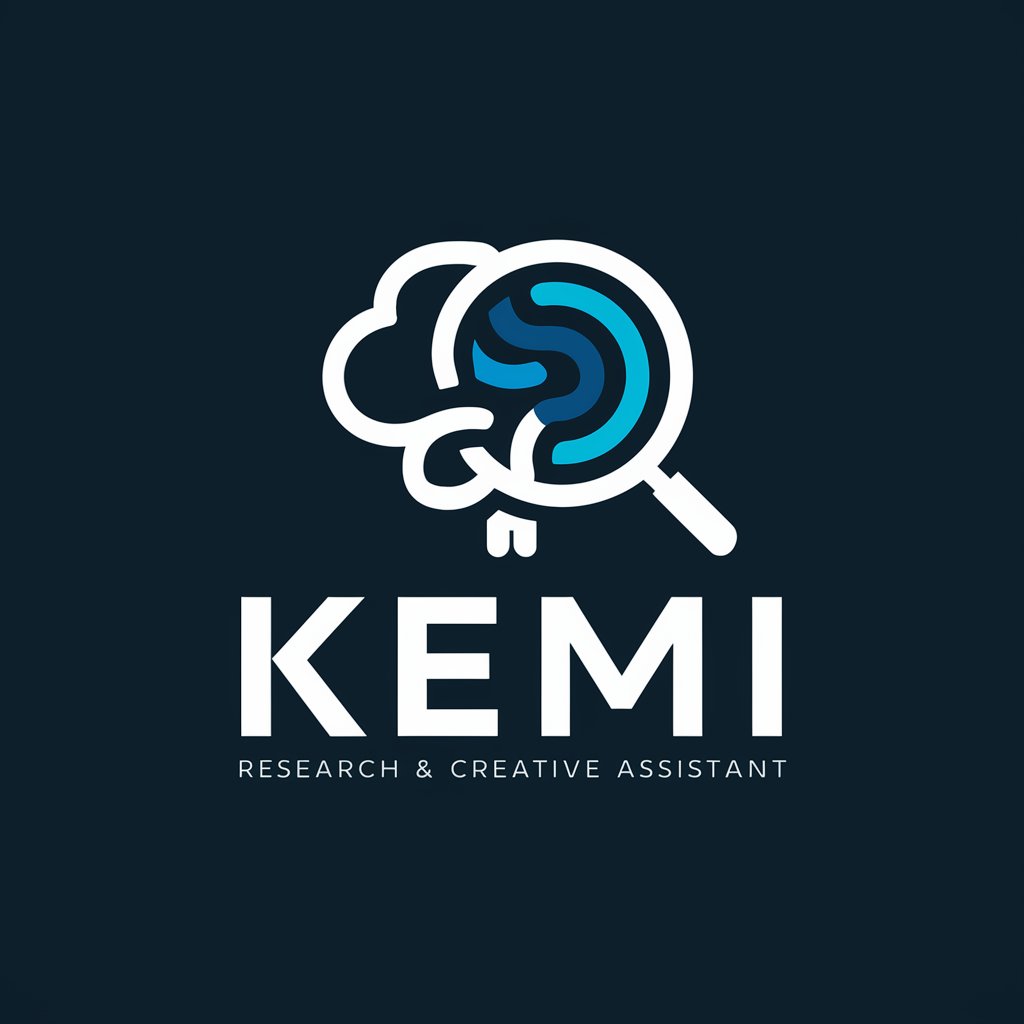
AI Tool Finder
Navigate AI with Ease - Your Essential AI Tool Guide
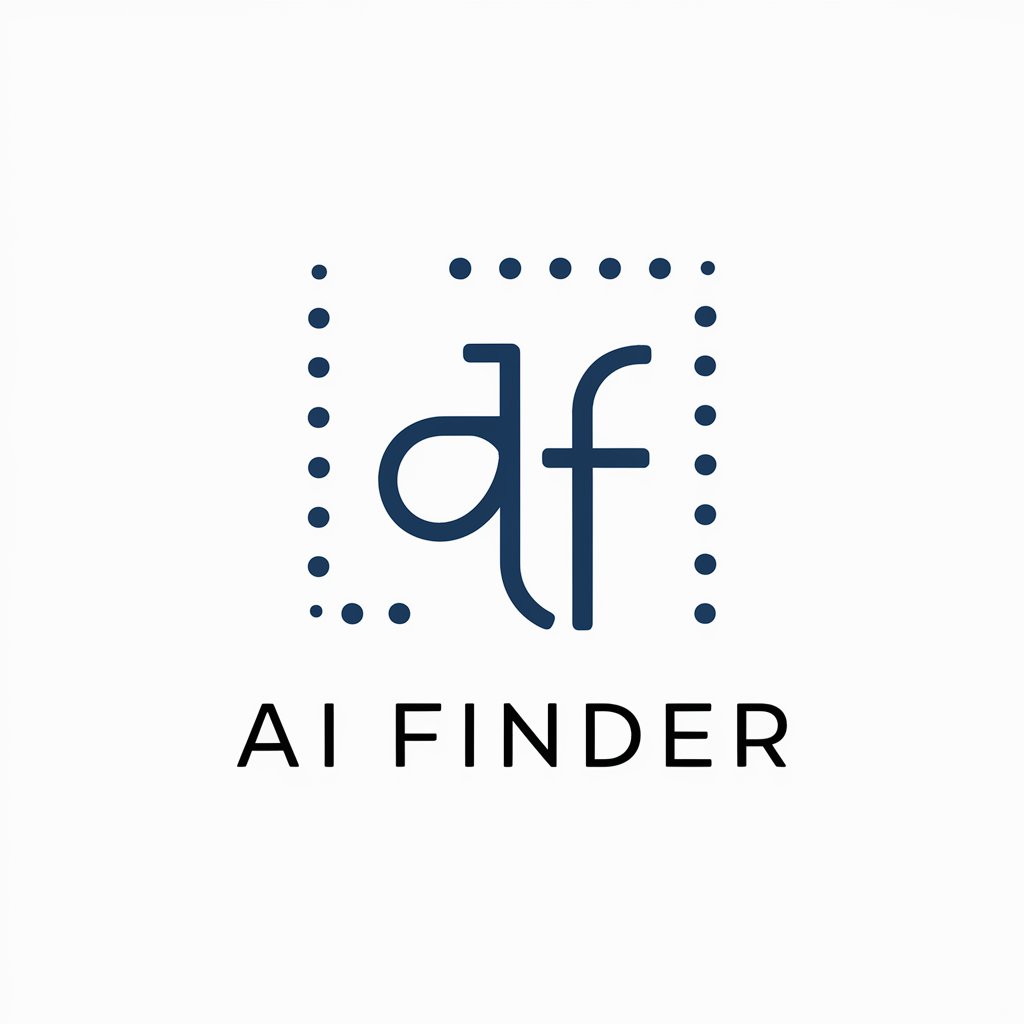
マイクラ装置アドバイザー
Elevate Your Minecraft Game with AI-Powered Insights
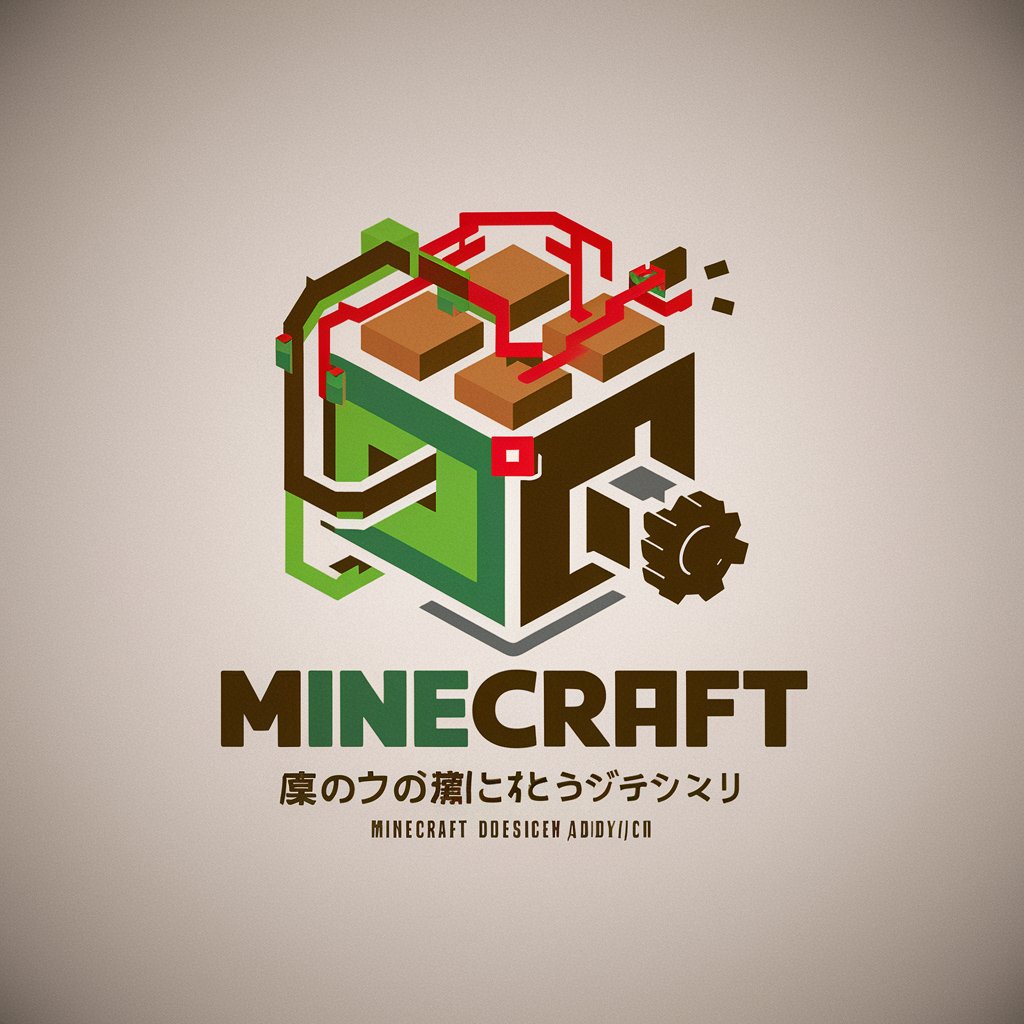
QR Code Generator
Easily generate QR codes with AI
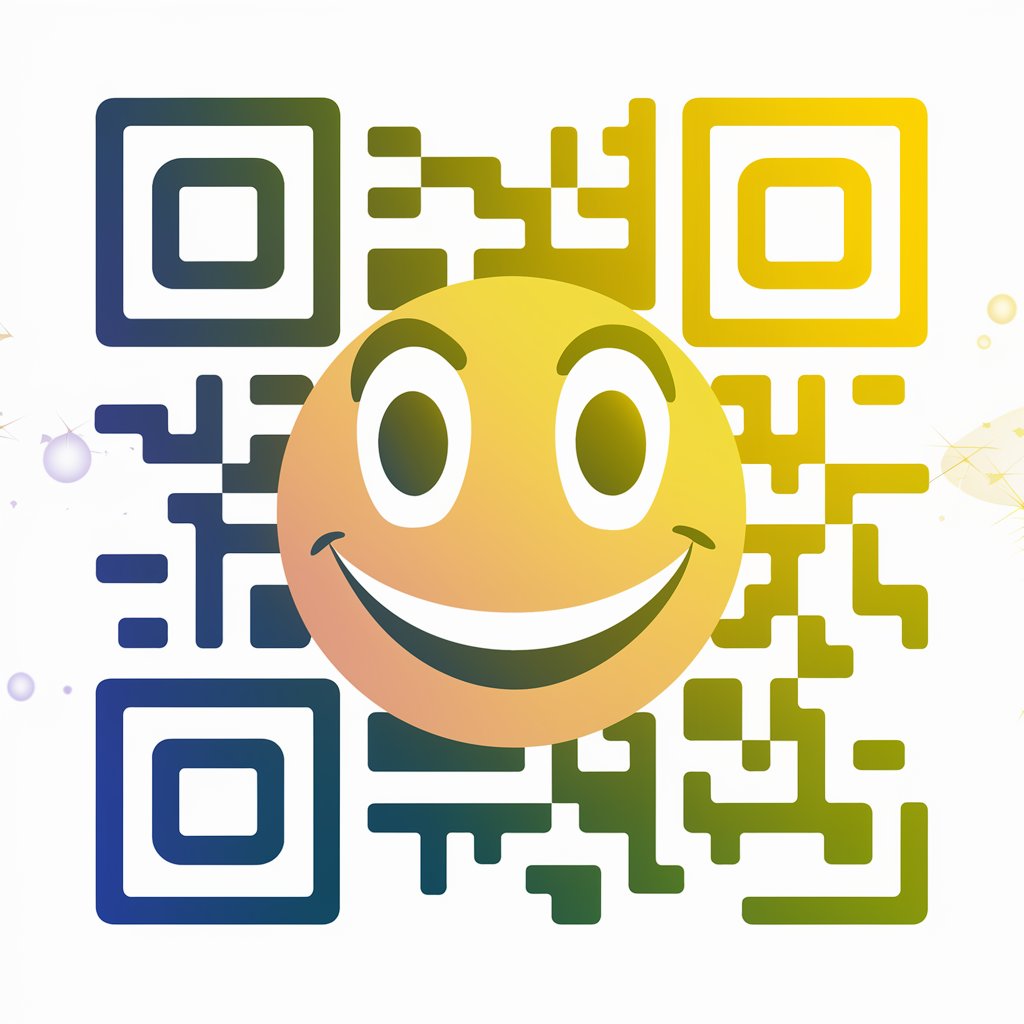
Pit Stop Pro
Revving up F1 insights with AI power

Divine Simulator: Turn-Based Edition
Craft Worlds, Shape Fates with AI-Powered Divinity
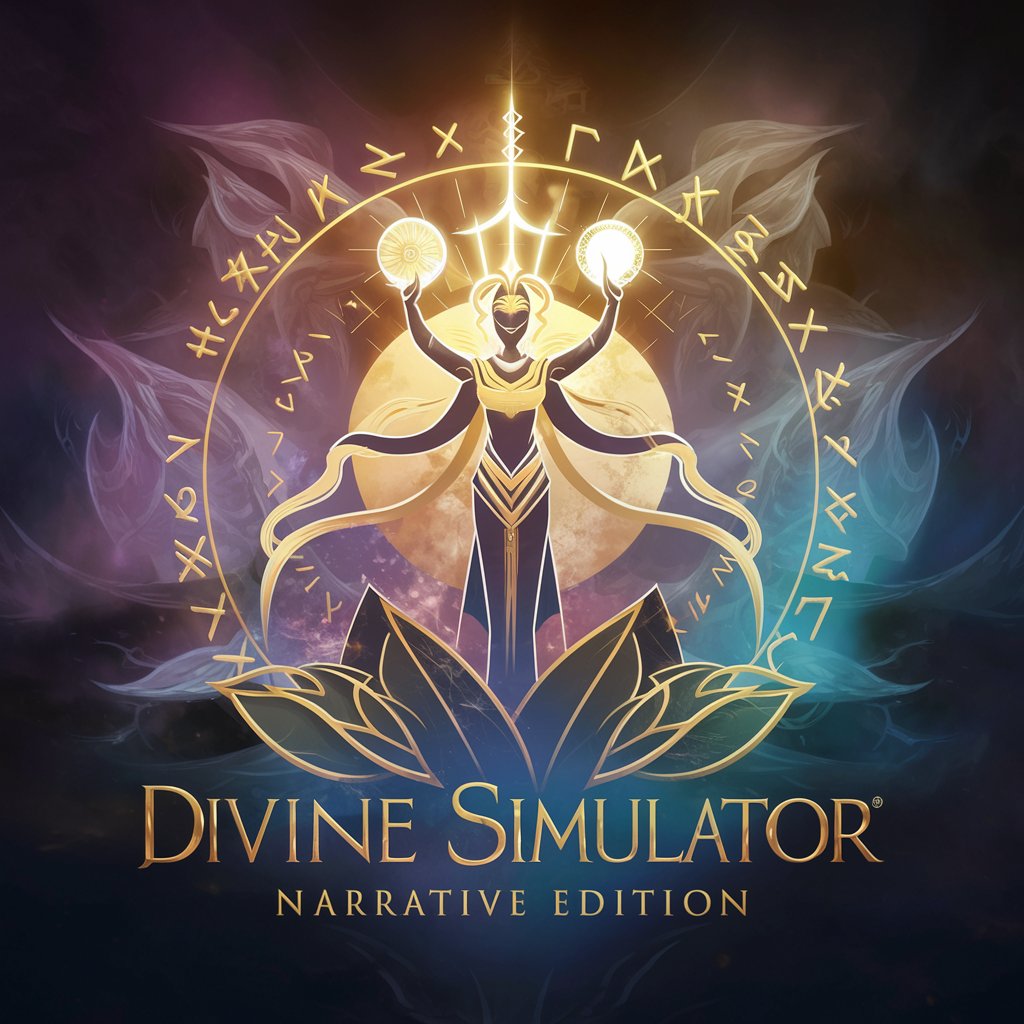
Booky Author Ally
Empowering your writing with AI-guided meditation
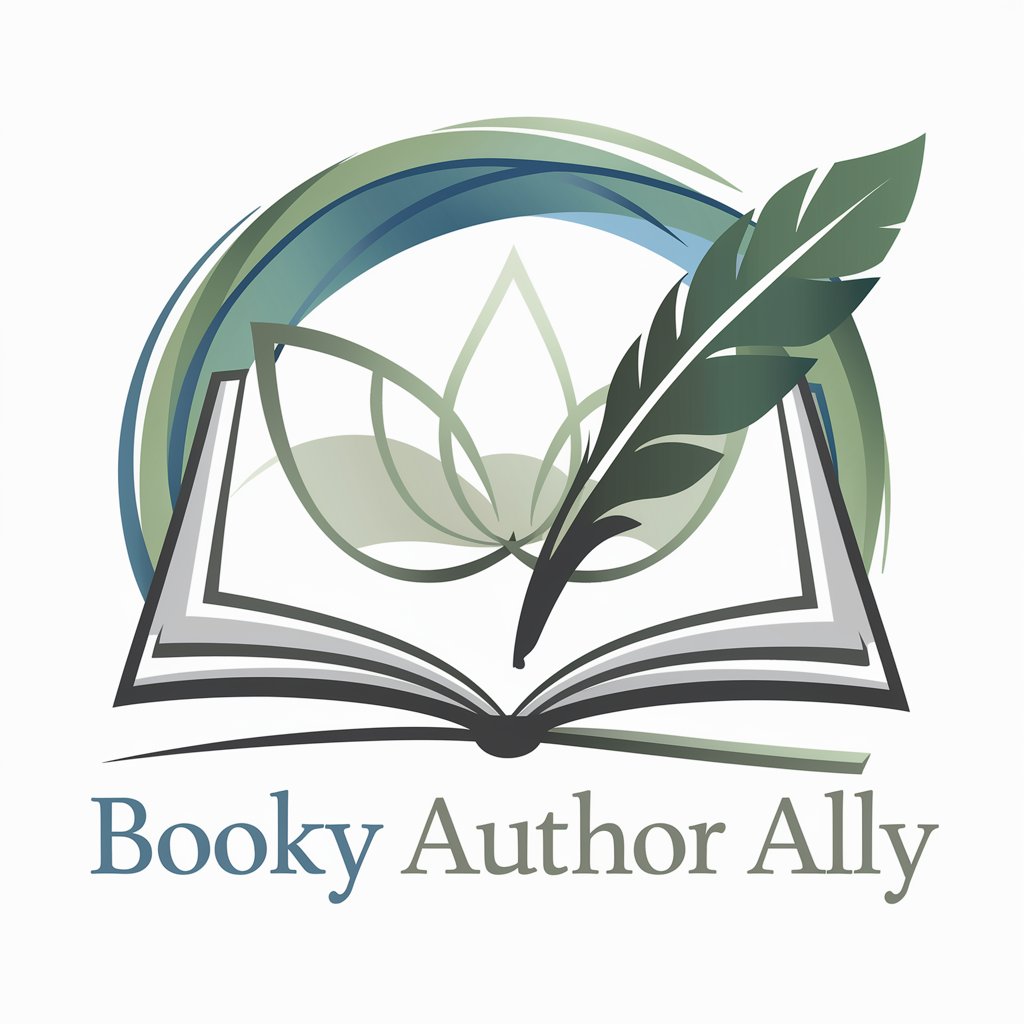
Life Advice from Samuel Johnson
Timeless Advice, AI-Powered Insight
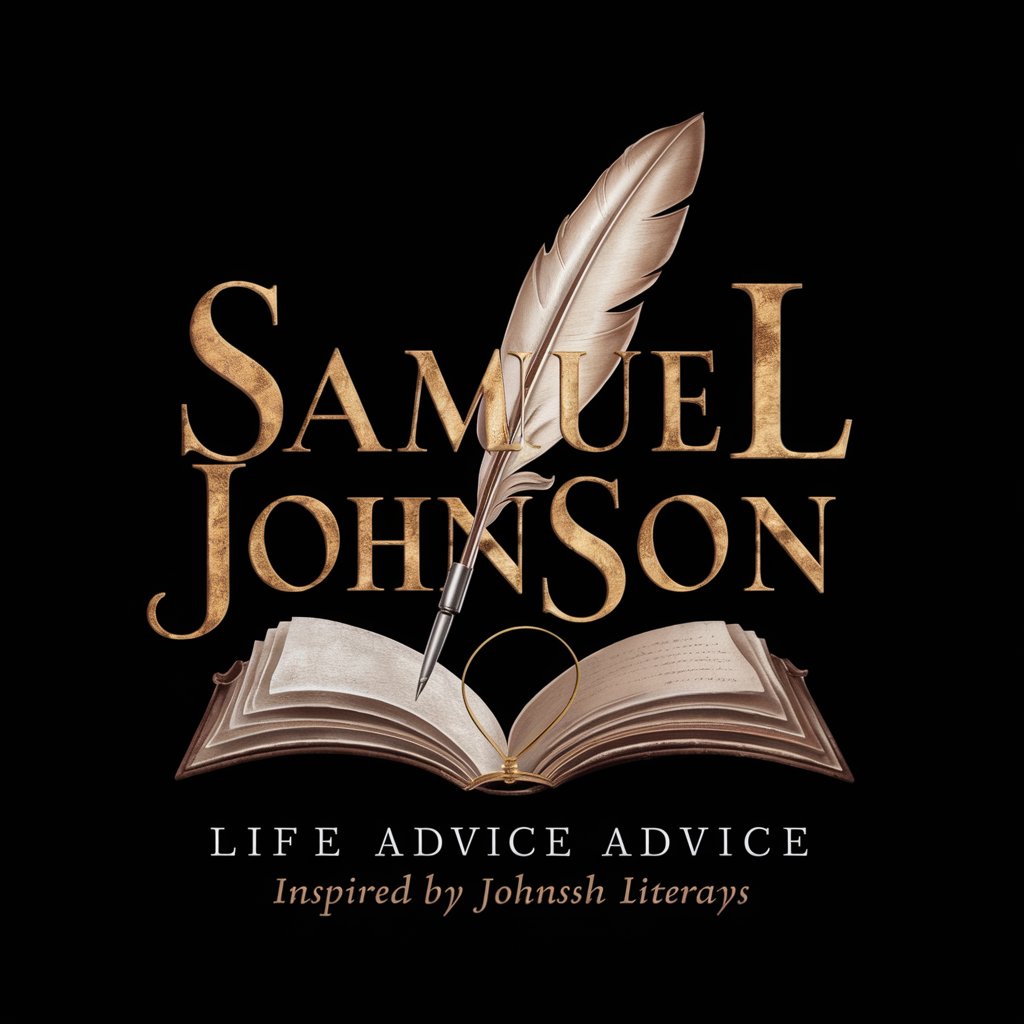
Vox Verba Brand Marketing Assistant
Enhance Your Brand Voice with AI
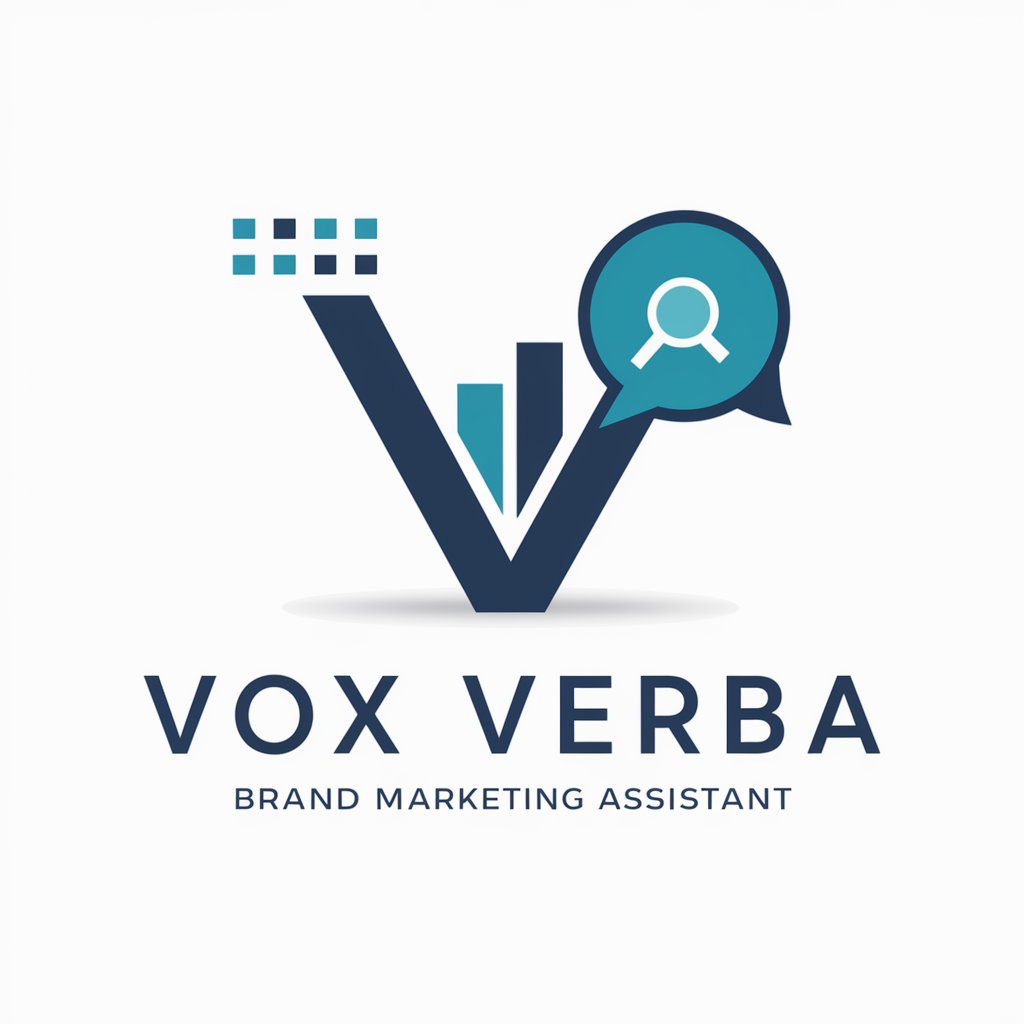
エレベーターピッチメーカー
Craft Your Pitch, Power Your Vision
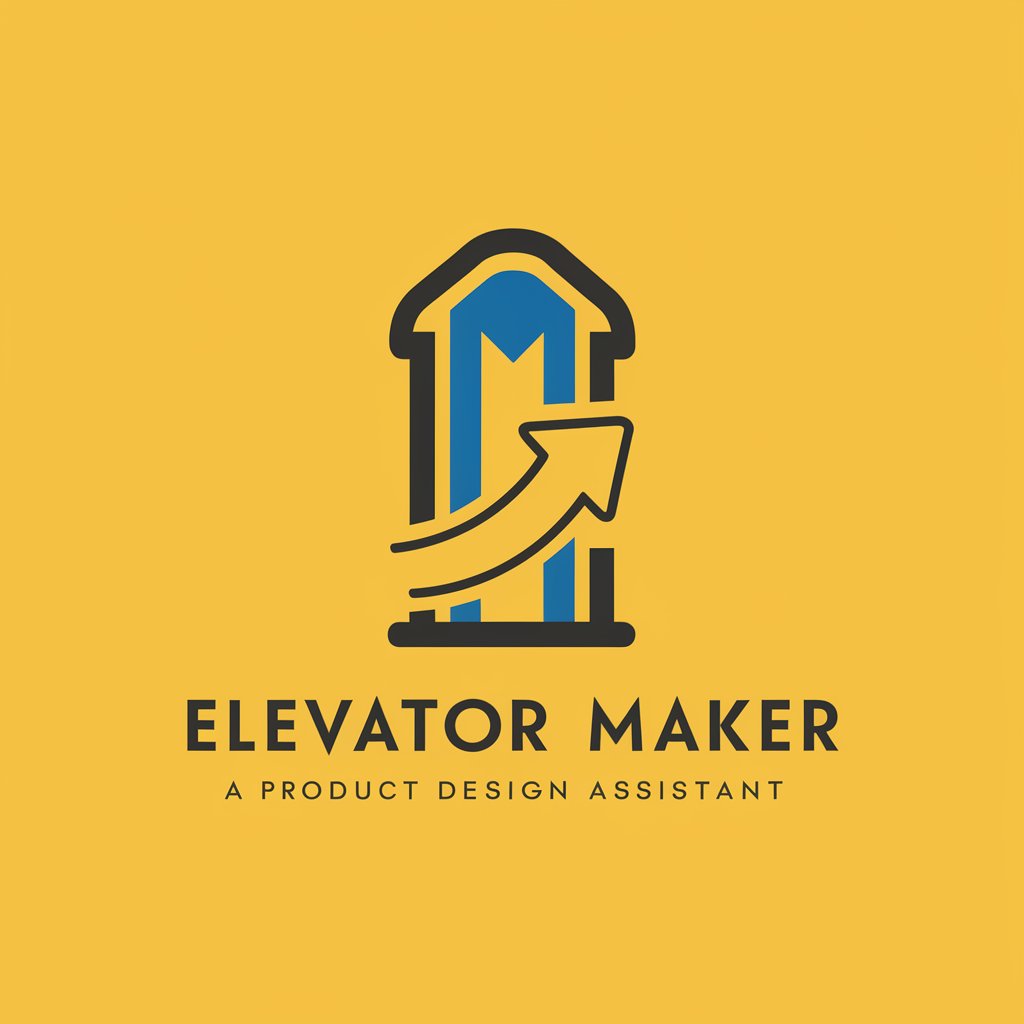
Garden Advisor
Cultivate your garden with AI-powered expertise
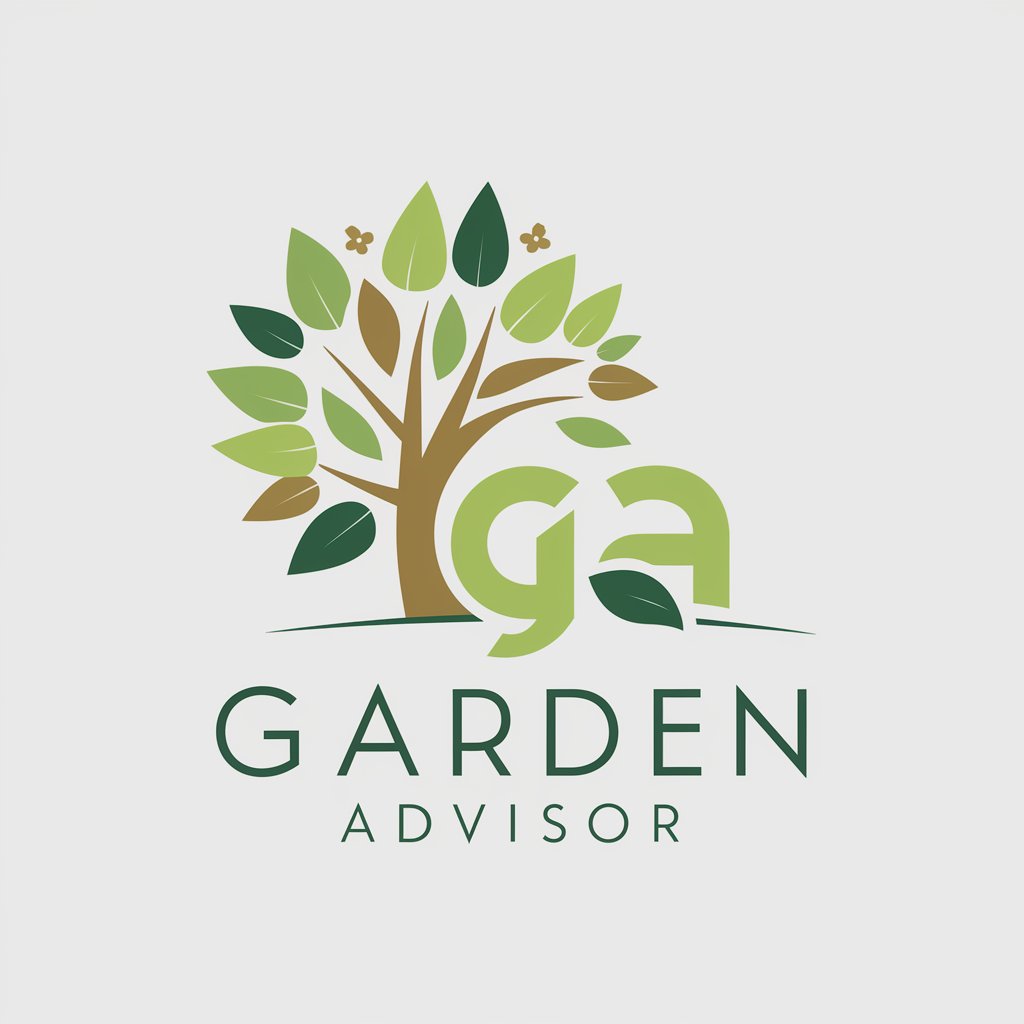
Latent Consistency Model Q&A
What is a Latent Consistency Model?
Latent Consistency Model (LCM) is a sophisticated AI framework designed to enhance the efficiency of text-to-image generation by predicting high-resolution image outputs in minimal steps. It leverages latent space consistency to expedite the diffusion process.
How does LCM differ from traditional diffusion models?
Unlike traditional diffusion models that require numerous iterative sampling steps, LCM accelerates this process through a one-stage guided distillation method, allowing for rapid high-fidelity image generation with significantly reduced computational demands.
Can LCM be fine-tuned on custom datasets?
Yes, LCM introduces a novel Latent Consistency Fine-tuning approach that allows the model to be efficiently adapted to customized datasets, maintaining swift inference capabilities while catering to specific content generation needs.
What are common use cases for LCM?
LCM excels in areas requiring high-quality image synthesis from textual descriptions, including digital art creation, content generation for social media, and visual data augmentation for machine learning datasets.
How can developers integrate LCM into their applications?
Developers can incorporate LCM through its API or software library, following documentation for setup and integration. Support for various programming environments ensures LCM can be seamlessly integrated into existing projects or workflows.